Maps vs. Ground Truth in Phoenix, AZ: Ready for Autonomous Driving?
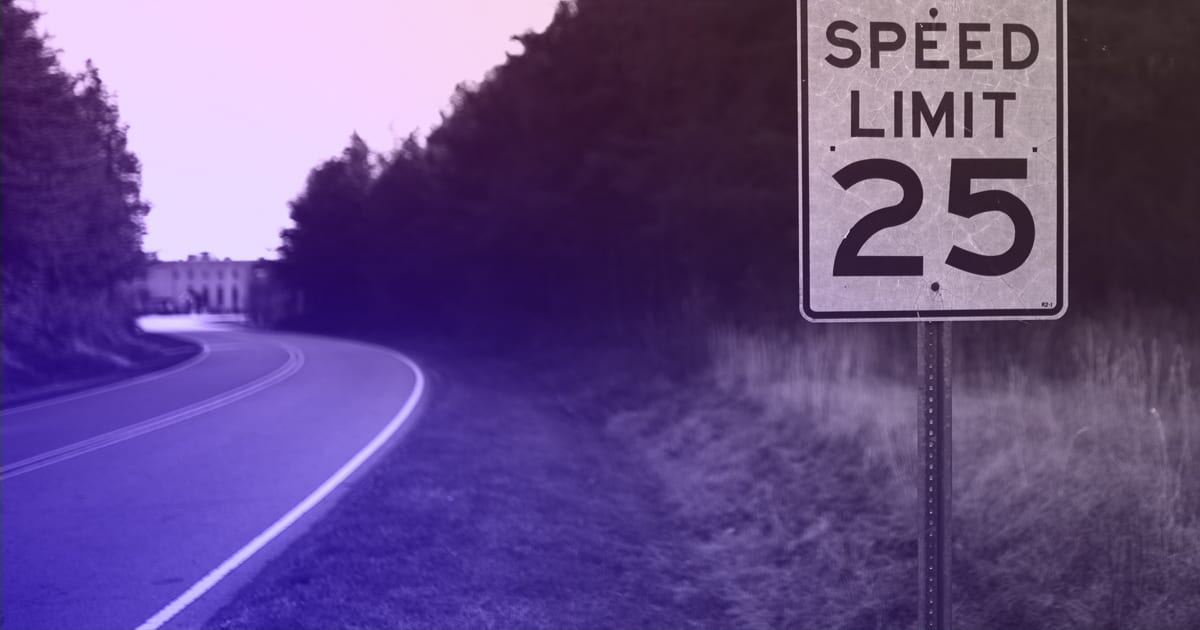
Comparing Nexar's Phoenix, AZ ground truth data with both City of Phoenix and OpenStreetMap (OSM) limit data shows that maps become stale. This post details the findings of our analysis for Phoenix, AZ speed limit signs. You can also read why this means we need to think about scalable map creation for autonomous driving, changing how we think about maps in this post by Eran Shir, Co-Founder and CEO of Nexar.
TL;DR
There’s a difference between what maps show and what actually exists in the real world. While humans can adjust to missing data, new (software-) map users, from AVs to driver assist functions (such as Intelligent Speed Assist), cannot. While some map layers, such as the base map, seldom change, additional map layers, such as road furniture (e.g. traffic signs), or lane information change more often. To ensure safety for AV applications, the data in all relevant map layers should be highly accurate, with any change quickly detected and updated on the map.
In this ground truth study we compared fresh crowd-sourced vision data about speed limit signs in Phoenix, AZ with pre-existing speed limit data from the City of Phoenix and OSM (OpenStreetMap). We wanted to check the accuracy of speed limit data vs the ground truth (which is required for AV applications), and infer the rate of change (or error) for road speed limits, which would help define how often map data should be updated or verified.
- Comparing our Phoenix, AZ ground truth data with both City of Phoenix and OSM speed limit data shows an error rate of 3-6%, meaning that speed limits posted in streets are not what appear in map data.
- This may be due to changes that happened after the data was inserted into the map, or errors in map creation, and correlates with our findings in Las Vegas, NV, based on Nexar’s vision network and change detection capabilities, the annual rate of street sign change is 2.5%.
- In the case of OSM, 47% of speed limits didn’t appear at all, pointing to a lack of coverage that may also exist with traditional map players.
- Crowdsourcing vision data to “see” ground truth speed limits - as an additional layer on a map, made for driver assist and AVs - is feasible, scalable and useful to ensure map accuracy and change detection.
- Assuming the speed limit layer for driver assist and AVs needs to be 99.5% or higher, as with many automotive applications, AV maps should be updated more often. Using the Las Vegas Nexar numbers shows that updating a map just once a year would only result in 97.5% accuracy.
Introduction
As the real world around us changes, maps are predestined to become stale. AVs need to understand the road, and need to become “aware” of any change on the road. Maps will need to reflect change so they can stay accurate, showing everything on the road from work zones to lane markings and road signs, such as speed limits.
While others have checked OSM’s applicability for rideshare, we wanted to look at its applicability to speed limit data, which is of special importance to the future of self-driving. Speed limits aren’t very dynamic, certainly less so than work zones which can change daily or hourly - but they do change.
By collecting crowdsourced data from our vision network and comparing it to OSM data and to City of Phoenix known road speed limits, we wanted to check the accuracy of speed limit data and its rate of change. Understanding the accuracy of both city-sourced data and OSM against the ground truth represents the rate of real-world change (or map error data) and how it impacts the accuracy of maps and their usability for autonomous driving. It also presents a roadmap for the future use of crowd sourced data to create better maps.
Printed-on-paper maps are destined to become stale at the exact moment of their publication, since the ground truth always changes. Mapping giants invest endless resources to update maps in an expensive and laborious process. Continuous coverage is not a viable option for traditional mapping since they either use expensive vehicles for mapping or rely on reports of change; if the data changes (a speed limit was decreased/increased) or is transient (a work zone) it likely won’t get picked up automatically. Since map elements don’t contain a time stamp it’s difficult to know whether they are accurate or not.
Historically, humans used maps mainly to navigate; they wanted them to tell them how to get somewhere, not to understand road rules. Yet, increasingly, maps are not only used by humans, but also by software. AVs and other automated driving scenarios (Intelligent Speed Assist, for instance) need a different kind of map - a map that is detailed, at lane level or better, with a high degree of accuracy, and that reflects transient changes on the road.
Autonomous Vehicles need maps that are precise and contain fresh data, at scale. Humans do not refer to posted speed limits on a map while driving, but software does. This means that the question of how much change happens in maps, and who uses it (software) is about to change how we think about maps and their freshness and accuracy.
In general, one can identify 4 layers in maps that AVs and driver assist apps will require:
- Basemap
- Lane semantics
- Road furniture
- Dynamic location-based services
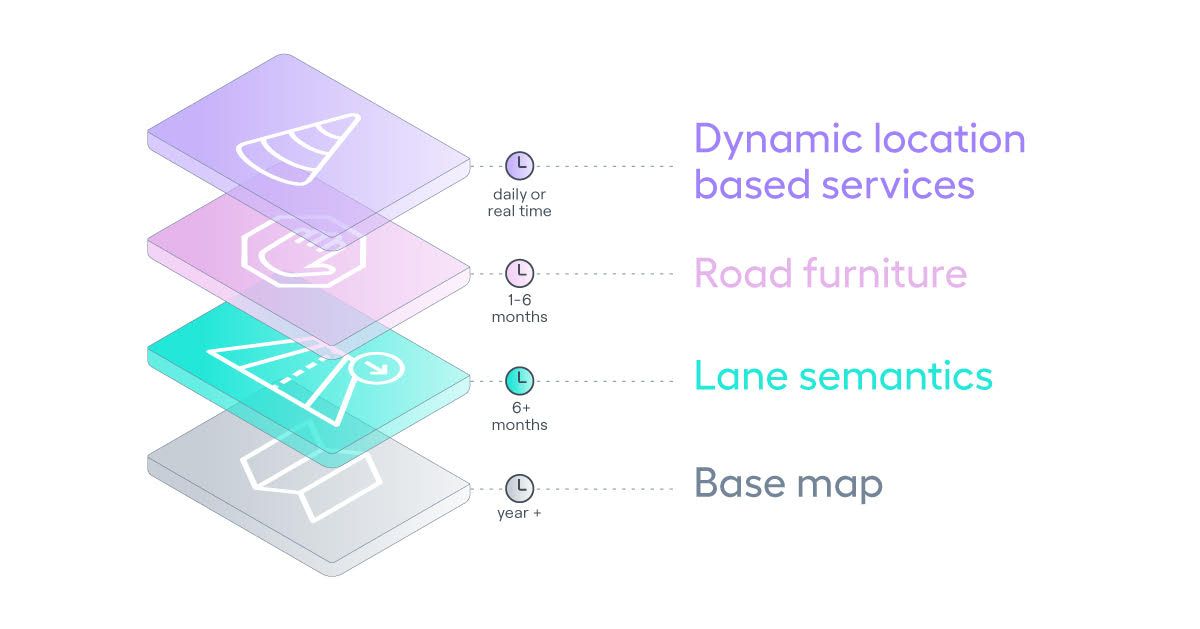
Findings
Comparing our Phoenix, AZ ground truth data with both City of Phoenix and OSM speed limit data shows an error rate of 3-6%, meaning that speed limits posted in streets are not what appear in map data. This may be due to changes that happened after the data was inserted into the map, or errors in map creation. This correlates with our findings in Las Vegas, NV, based on Nexar’s vision network and change detection capabilities, that the annual rate of street sign change is 2.5%.
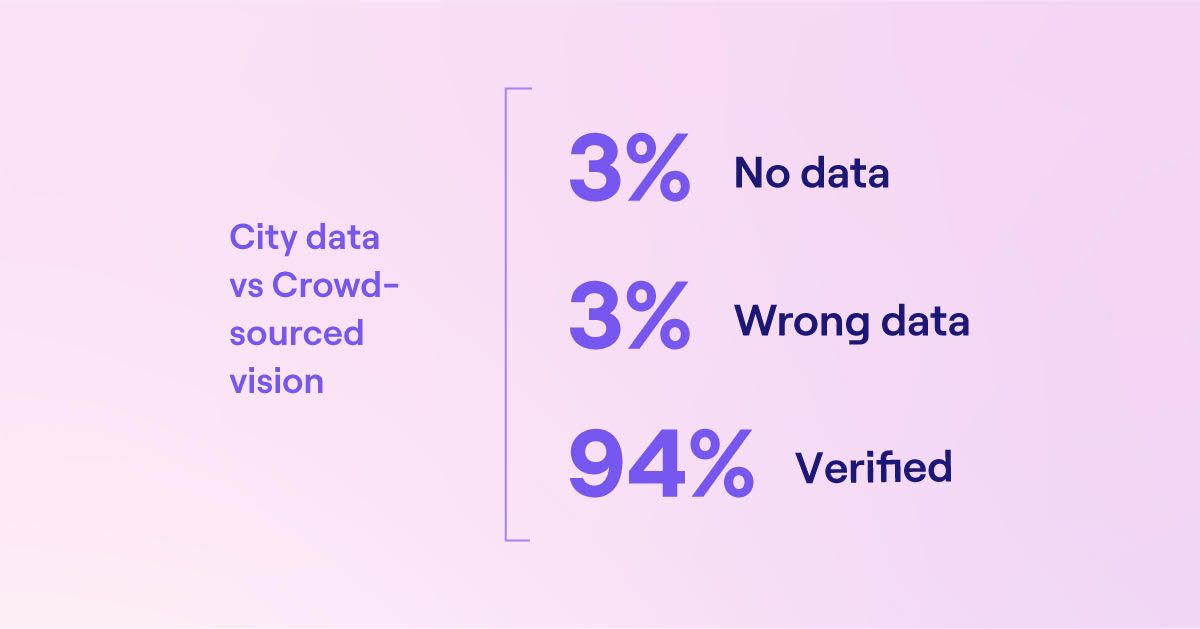
Additionally, in the case of OSM, 47% of speed limits didn’t appear at all, pointing to a lack of coverage that may also exist with traditional map players.
Crowdsourcing vision data to “see” ground truth speed limits - as an additional layer on a map, made for driver assist and AVs - is feasible, scalable and useful to ensure map accuracy and change detection.
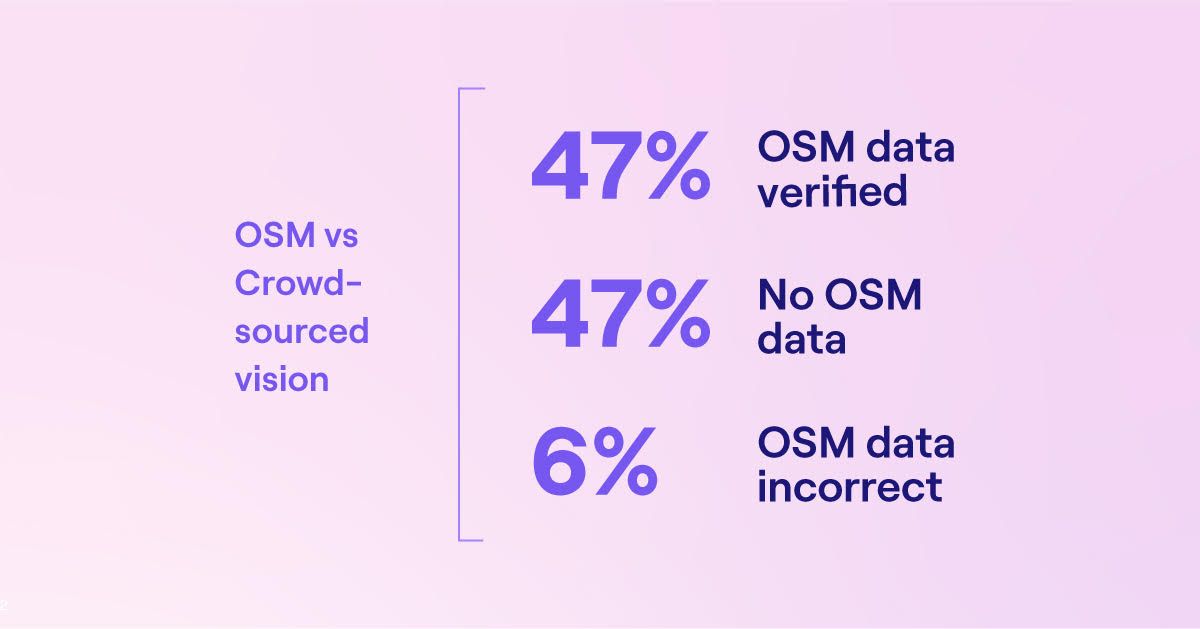
Assuming the speed limit layer for driver assist and AVs needs to be 99.5% or higher, as with many automotive applications, AV maps should be updated more often. Using the Las Vegas Nexar numbers shows that updating a map just once a year would result in 97.5% accuracy.
To read how we arrived at our conclusions, download the report here.
Conclusion
In the era we are entering, the maps of yesteryear just won’t cut it anymore.
As maps move from being a convenience to becoming the cornerstone of ADAS and AVs, the levels of precision, freshness and completeness that are required grow exponentially, and as a result, the core business process of map creation needs to be overhauled to better reflect the ground truth.
This report used data from just one month, October 2021. This means that using crowd sourced vision data to map speed limits is feasible as a way of effectively auditing map data and changing it to reflect the ground truth.
Assuming the speed limit layer for driver assist and AVs needs to be 99.5% or higher as with many automotive applications, maps should be updated more often. Even using the Las Vegas Nexar numbers shows that a once a year map update would only result in 97.5% accuracy.
In short, to build the maps of the future - the digital twin of the physical world- we need to embrace crowd-sourced vision and change detection. At a change level of 2.5 to 6% questions of how to ensure maps are fresh should be taken seriously by the industry as a whole.
Download our full report to see how we arrived at the conclusions here