Announcing AI-Powered Image Retrieval method for Better Localization in Cities
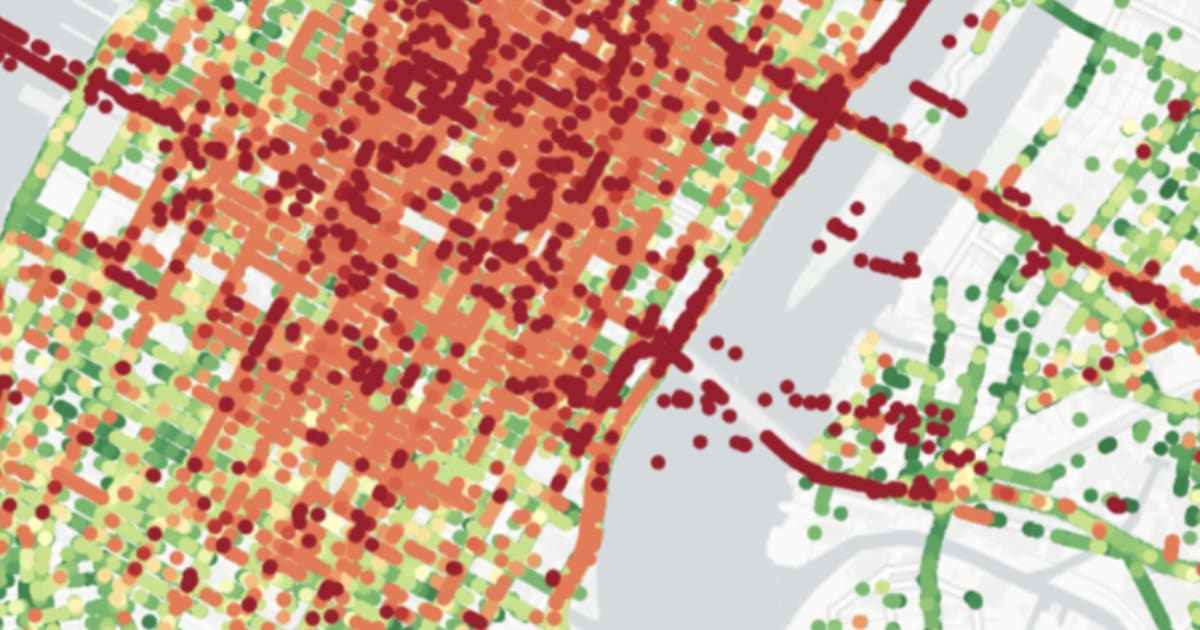
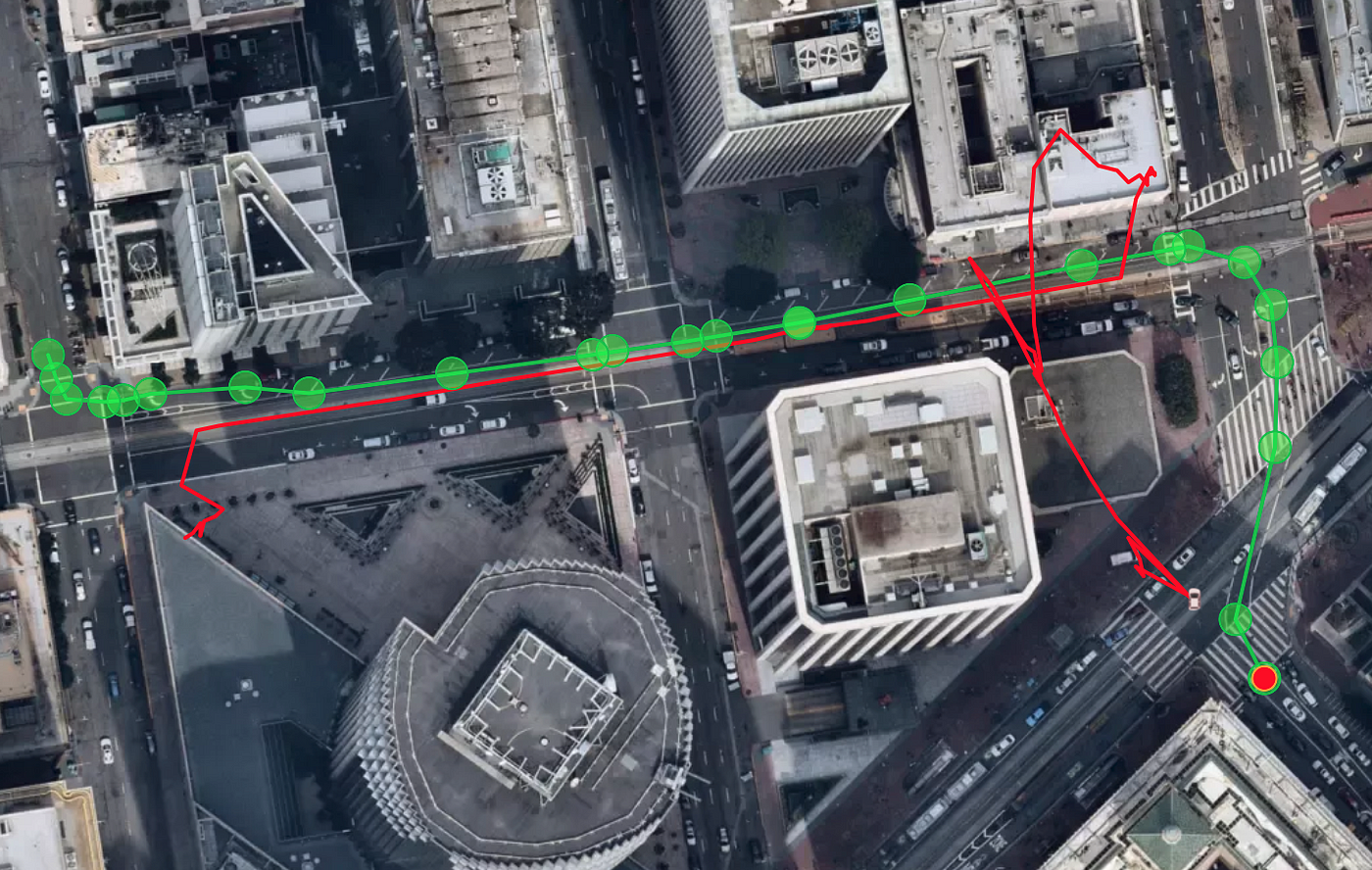
Today we are very excited to announce that Nexar has developed a new, AI-powered visual localization method that greatly improves GPS location accuracy in challenging urban environments. We’re presenting this new method today at the Computer Vision and Pattern Recognition Conference (CVPR), the premier annual computer vision event.
Here are more details…
What is localization?
Basically, understanding the precise location of an object in its environment. So, for example, when we drive a car from point A to point B, we usually use GPS and mapping applications to know where our car is on the road. But in dense urban environments like New York or San Francisco, GPS signals are often blocked or partially available due to high-rise buildings, resulting in GPS errors of 10 meters or more. This poses a critical challenge when it comes to safety applications such as what Nexar is building.
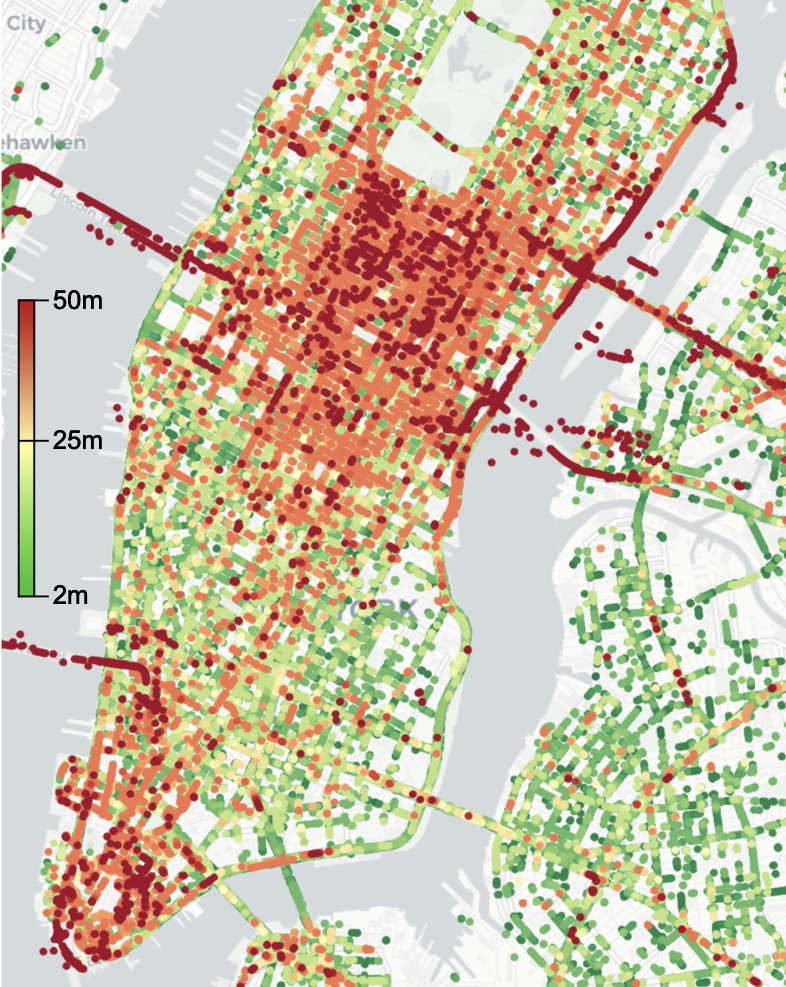
Why is that?
Because at Nexar, we’re building a network of connected vehicles that are alerted to upcoming hazards beyond the line of sight — collisions, closed lanes, traffic. These alerts come from others on the road ahead. In order to deliver these critical alerts, we need to know the precise location of the vehicles in the network. And we can’t afford to be off by 10 meters.
Got it. So what’s different about Nexar’s localization approach?
Technically speaking, we’ve developed a scalable approach for accurate and efficient localization that leverages Nexar’s continuously growing database of fresh road imagery observed by its network.
Explain.
We use a large-scale dataset comprised of billions of anonymized images from more than 400 million miles driven on the Nexar network. We then trained a deep-learning model to encode images according to their geo-location. This compact encoding allows efficient retrieval of images with similar geo-location by identifying road anchors that are relevant for localization (e.g., traffic-lights, road signs, buildings, etc.) and ignoring temporary things on the road (e.g., cars, pedestrians). Our method, based on constantly refreshing data from the Nexar network, solves for the challenges presented by other mapping companies, such as Google, which can use a vast Street View image repository, but lack the minute-level freshness that Nexar provides.
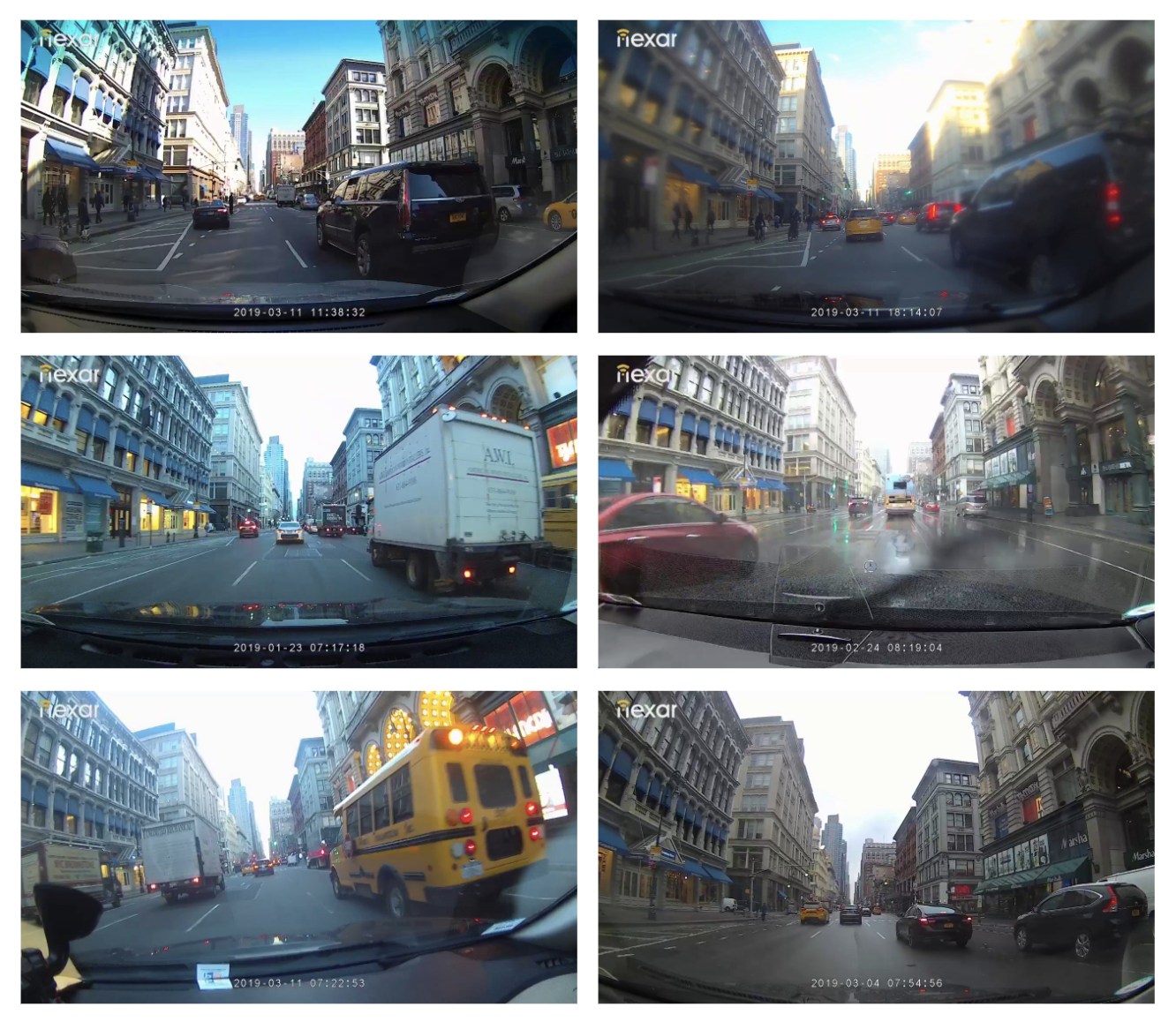
Does it work?
Yes. Our experiments confirm that this localization approach is very effective in challenging urban environments like New York or San Francisco and improves the GPS location accuracy by an order of magnitude.
So…how does this affect me?
For one, this method will be used to deliver critical real-time alerts to Nexar users, like warnings about dangerous intersections and collisions on the road ahead. But the benefits can extend much further — this approach could one day allow autonomous vehicles to reliably navigate cities. It is faster and far less expensive to teach a computer to drive like a human using a camera for eyes than by using structure-based techniques such as LIDAR, which are limited in scale and expensive to compute.
Want to go a level deeper? Here’s our paper detailing our research and findings.