Monetizing Alt Data Products, Part 1
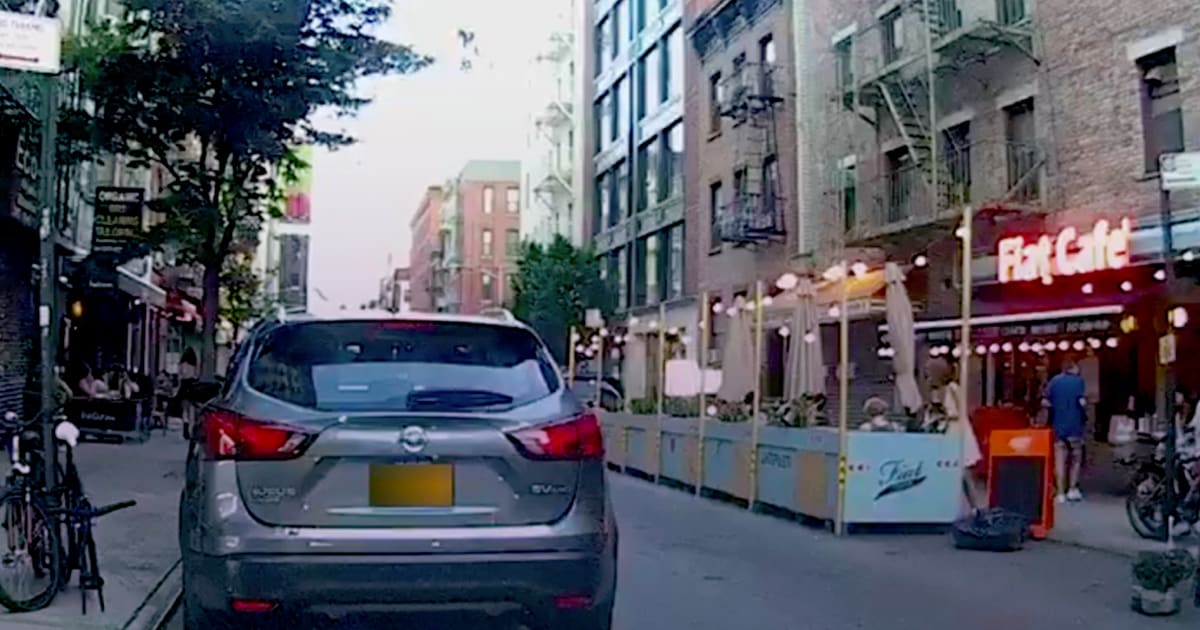
The market for Alternative Data has evolved dramatically in recent years for both buyers and sellers. I’ve been fortunate to witness this movement first hand at three separate companies. Though each was leveraging differentiated data & processes, the challenges were similar. I’m constantly reminded of these lessons as we try to build a new category for ground-level imagery at Nexar.
In the coming weeks, I’ll reflect on the now-widespread adoption of Alt Data and what this means for those trying to monetize scalable data products. In this first post, I look back on my time in the “boom days” of Credit Card data adoption.
Part I. Early Adoption of Credit Card Data (2015–2017)
The “Wake Up Call”
I spent the first four years of my career in Financial Services, as an options trader and then as an adviser at an investment firm. As most 25-year olds can relate, this was the stage of ‘not knowing what I didn’t know’. Amidst the firehose of information, one trend seemed clear — “stock pickers’’ were falling out of favor, while low-cost index funds and robo-advisors were taking off. I quickly surmised that the ‘ability to consistently pick stocks better than the crowd’ was the result of: 1) an abundance of luck; 2) unhealthy doses of confirmation bias; and/or 3) access to information that others don’t have (which to my knowledge was rare).
Just when I was polishing my resume for openings at the latest robo-advisor, I stumbled upon something startling: A company called 1010data had procured anonymized credit card transactions for millions of US consumers. The source was another tech firm that aggregated financial information for consumers (allowing them to see all of their accounts in one app). Cue the disclaimer: the data was cleansed of any Personally Identifiable Information (PII) and required opt-in consent from the user. Compliance aside, here’s where it got interesting.
1010data processed, tagged and restructured the data, then resold it to hedge funds. The reports, containing daily-updating sales estimates for over one hundred US consumer businesses, allowed these investors to get a near real-time view of a company’s earnings, well before they were publicly reported.
This surely qualified as “access to information that others don’t have”. Maybe stock-picking…wasn’t dead yet?
The “Why”
Less than a month later, I joined 1010data’s Equities group. At the time, we were basically the only credit card data provider in town. There were a handful of early adopters in the investment community, but by-in-large, credit card data was a niche research product in a market with no shortage of skeptics. We still had to give people the answer to “why” — “why do you even exist, and why do I care?”
“Is the sample size big enough? How accurate can it be if it’s only representing 1% of total consumer spend?” I spent the first year trying to convince people of what amounted to an obvious ROI. “Yes, it’s expensive. No, it’s not an exact science….but the cost is a small fraction of the returns that can be tied directly to accessing our data.”
This wasn’t spin. With only a handful of customers accessing this information, the potential gains were borderline outrageous. Imagine that following early rumors of a food health issue at a small number of locations you could actually see data confirming a precipitous drop in Chipotle’s sales in 2015. Within a year the stock’s price was cut in half. Before you say the sell-off was clear-and-obvious, take a look at where sell-side sales estimates were in the few quarters following the first outbreak. Hint: “experts” forecasted much higher growth than our credit card data had implied.
Better yet, what if you could see in the data an exponential rise in customer acquisition for Amazon Prime in 2015, along with rock solid customer retention, only to see the stock double in the next year? And this isn’t to mention the run-of-the-mill “death of brick and mortar retail” that some folks were privy to just a little bit sooner than the crowd.
This isn’t to say that trades couldn’t be made in the absence of our data. It IS to say that there are cases where the data was practically PRINTING money for customers — like tens of millions on a single position — for a data set that cost a small fraction of that. If anything, the price tag was lower than it’s worth, mainly because it was an immature market with little precedent and a lack of institutional awareness around our product. Soon enough, we reached a tipping point as word spread in the hedge fund community of a single data set with the promise of outsized returns. We signed dozens of new customers — and pricing had increased.
With success came a new and entirely different set of challenges.
The “How”
Inevitably, more funds with access to the same information meant less drastic price corrections when companies reported. More money was on the right side of the trade before earnings, so less money flowed back in the other direction. While we were making the market more efficient, on a more real-time basis, we were also eliminating the “easy money” trades that led to our earliest customers’ largest returns. These headwinds were ultimately a sign of market maturity. Instead of convincing people “why” to use our data, we were now in a position where we had to show them “how” to use it in new ways.
First, users needed to understand the nuances of the data and how it was processed in order to know when they might be seeing a false read based on a data issue. When dealing with millions of individual transactions across hundreds of merchants, we could put processes in place to proactively detect most — but not all — issues. We couldn’t fool-proof the data but we could educate our clients on how to spot relevant and potentially misleading anomalies.
Next, we needed customers to extract more proprietary insights. Instead of focusing solely on whether a company will “beat or miss” in the next quarter, make a bet on a longer-term, directional thesis. For example, an online retailer may have accelerating sales growth for several years and credit card data implies that they’ll beat expectations yet again. At the same time, their more recent customers spend more of their money on competitive brands, on top of having higher CAC. One client sees strong CC sales this quarter and buys the stock. Another client, with a longer time horizon, sees the troubling cohort analysis and waits until after an earnings pop to short the stock. Two customers with opposing views on a different time horizon — and they’re both making money with help from the same data.
In a more extreme example of leveraging the data in different ways, we can look to the shift in usage from early adopters. Instead of the “easy money” trades of 2015, customers were now mining the data for “data issues” and using that to trade “against the crowd”. For instance, when Dillard’s POS systems started to label all transactions as “Shoes and Accessories’’ (at the time difficult to proactively capture as a vendor) many saw decelerating Dillard’s sales and expected a negative earnings surprise. A handful of savvy funds noticed the change in the POS labeling early enough to correct their data — and made money betting against the most basic interpretation of our data.
The “Many”
By 2018, the credit card data landscape had changed dramatically, due in large part to increased competition. The source provider of 1010’s data saw the success of the partnership and decided to increase their “channel reseller” ecosystem. Several start-ups wanted a piece of the action — and the technology required to wrangle multi-billion row tables (i.e. advances in cloud, warehousing, processing & AI/ML) — was far cheaper and more accessible than it had been just a few years prior. This has some obvious pros and cons for providers.
Increased competition can lead to innovation, which can lead to better data products. When 1010 was the “only shop in town”, in spite of great technology & people, it’s hard to argue that our greatest asset wasn’t exclusive data access (the “holy grail”). Now that there were four providers with access to the same raw data, we had to shift our strategic focus. We were incentivized to expand coverage, build an open platform for flexible data delivery across fund types & develop better processes for proactively “QA-ing” the data to avoid potential data issues.
Despite our best efforts, the fact remained that we no longer had pricing power. When a market has several marginally differentiated solutions (with no data exclusivity), vendors will compete on price. “Give the data away for free today”, in the hopes of upselling stickier customers tomorrow. The market price adjusts. On top of that, each new customer seemed to have higher expectations than the last. While our earliest users understood the potential pitfalls (and subsequent risks), it was the tail-end of the market that seemed to expect guaranteed returns.
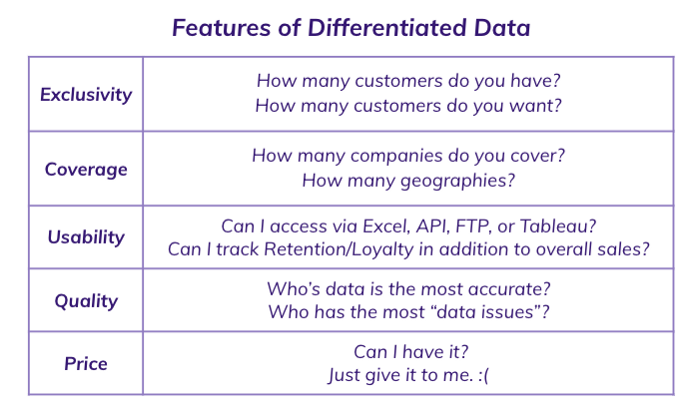
Lessons Learned
In just a few years, we went from “why would I even consider using this?” to “why would I use yours (and not ‘theirs’)” and all the way around to “I don’t want to use this because everyone and their Uncle uses it”. Despite the chaos, there were some clear & convincing takeaways.
- Exclusivity is King… but exceedingly rare.
- Early adoption is a major hurdle — but broad adoption (and commoditization) can happen fast.
- Commoditization can lead to innovation as vendors are forced to compete on coverage, quality & usability.
- The data “edge” isn’t necessarily gone — it shifts from data access to data usage (processing, infrastructure, data science & analytics).
- The market doesn’t naturally (or efficiently) lead to optimal pricing, especially with respect to novel data sets.
- Not all customers are created equally.
I’ll dig deeper into these lessons in my upcoming post, which will focus on the mainstream adoption of Alt Data, why I wanted to expand outside of Finance and the ways in which Nexar’s product development & commercial strategy are informed by the past.
For more information on Nexar’s multi-trillion image repository, reach out to me at jack.killea@getnexar.com.