Introducing BDD100K: The World’s Largest Driving Dataset
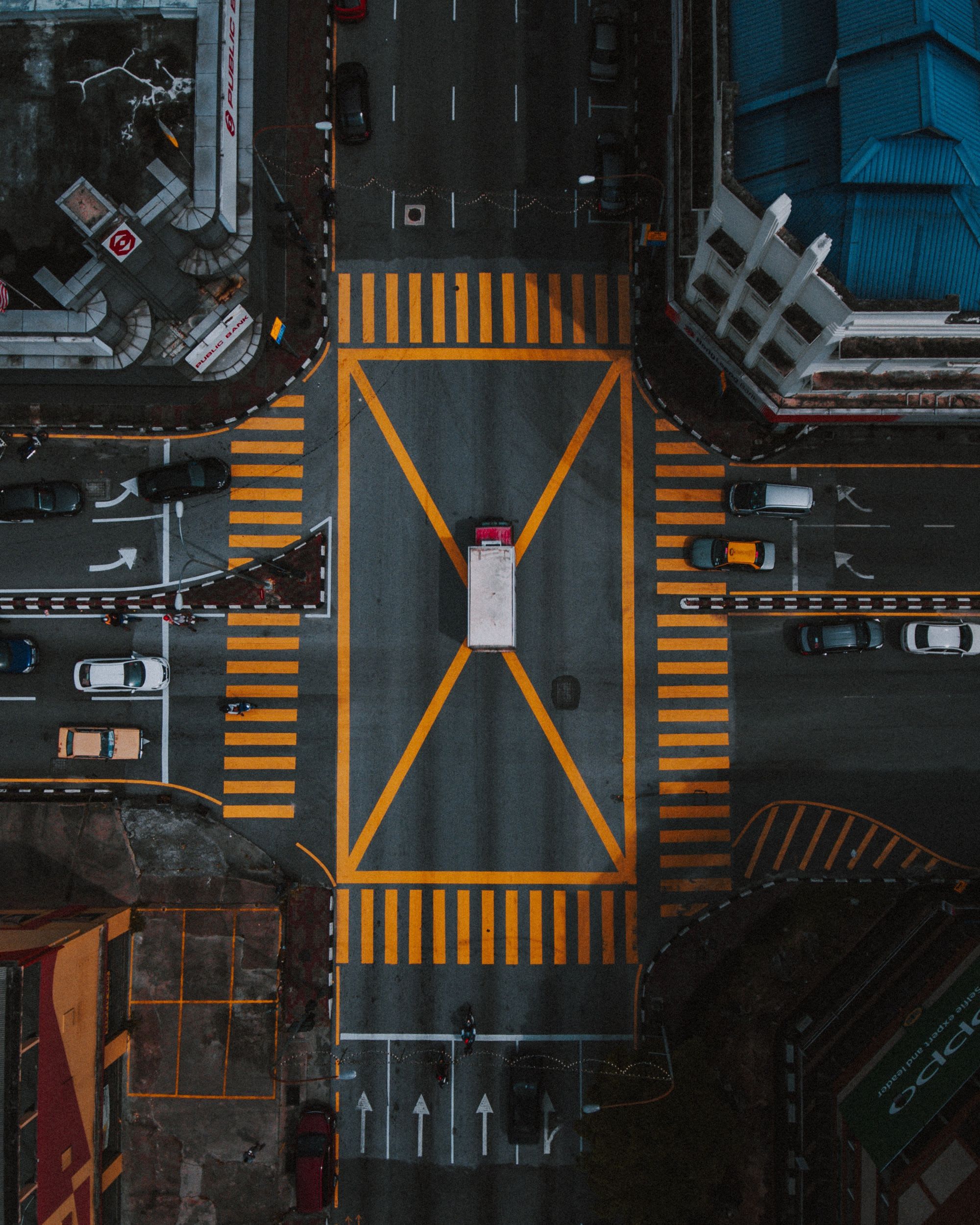
At Nexar, we invest a lot of time in learning about the world’s roads, driver behavior and what happens on the road on a daily basis. That’s because we are building a safe driving network and understanding our roads is an important step in creating a reality with no car collisions.
At Nexar, we invest a lot of time in learning about the world’s roads, driver behavior and what happens on the road on a daily basis. That’s because we are building a safe driving network and understanding our roads is an important step in creating a reality with no car collisions. To date, drivers using Nexar have driven more than 150 million miles. The anonymized footage of these driven miles helps us and other companies, researchers, and institutions develop accurate automotive perception and decision control models.
That’s why we are excited about the release of the world’s largest driving dataset called BDD100K by the Berkeley DeepDrive Industrial Consortium in partnership with the Berkeley Artificial Intelligence Research Lab of the University of California, Berkeley.
Using driving data collected by the Nexar network, the BDD100K dataset is the largest and most diverse open driving dataset for computer vision research, consisting of 100,000 videos. What makes this dataset so unique and valuable for researchers is that it’s large-scale, diverse (in terms of location, weather and time of day), and captured on real world roads. These characteristics are particularly important for creating robust perception algorithms.
The release of BDD100K is a key milestone in autonomous and assisted driving, by giving access to academic and industrial researchers a large Volume of annotated driving data with unparalleled Variety. Combining Volume and Variety of driving data is what truly characterizes Nexar’s network, and it is critical to make deep automotive drive technology a reality.
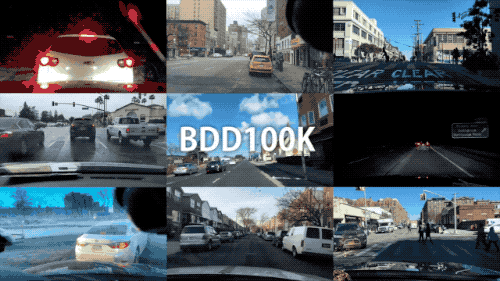
Our friends at BDD/BAIR have extensively annotated the driving data to provide an impressive dataset for researchers across the world. These annotations include:
- Bounding boxes for common objects found on the road like traffic lights and signs, buses, bikes etc.
- Two types of lane markings- vertical, which are along the driving direction of the lanes and parallel, which indicate where vehicles in the lane must stop.
- Drivable areas, which indicate where on the road drivers can actually drive. There are two types of drivable areas- direct drivable, which indicates that the car has priority on the indicated road and alternative drivable, which indicates that the car can proceed but should be cautious because other cars may have priority.
- Full-frame instance segmentation.
We are really eager to see how the BDD100K dataset will contribute to advances that are being made in mobility and more specifically, road safety. Interested in accessing the dataset? Check it out here. Want to join our team? Take a look at our openings here.