How Will Integrated Car-Cameras Change Vehicle and Fleet Insurance?
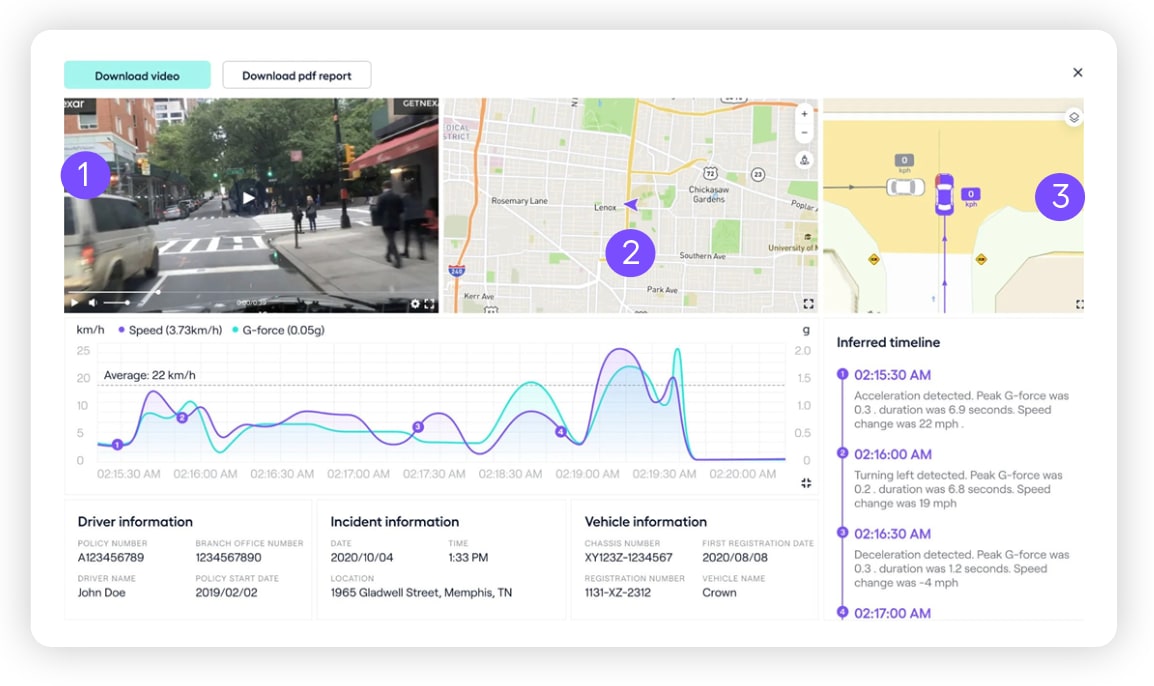
In the near future all cars will collect vision data from front-facing cameras. What does this mean for insurance and claims adjustment and resolution? What can we learn from current dash-cam AI solutions?
Introduction
A study by Autopacific shows that 70% of all new car buyers would want their next car to come with an integrated dash cam. Indeed, industry analysts predict that by 2024 most new cars will come jam-packed with several cameras inside. Those cameras won’t just show what’s around the car (for parking assist, for instance) but will also be connected, using LTE or 5G, for real time mapping, safety and other applications. Connectivity will be two-way: data generated by cars will be consumed outside of them, and data coming from outside the car will be used in it. The “connectivity budget”, or, in simpler terms, the bandwidth required to power the connection from the cameras to the cloud, will also be managed by different technologies, ensuring the feasibility of these solutions.
All this may sound just great for the future of driving, but what does this mean for car insurance, whether for consumers or fleets? What will be done and can be done with all this visual information?
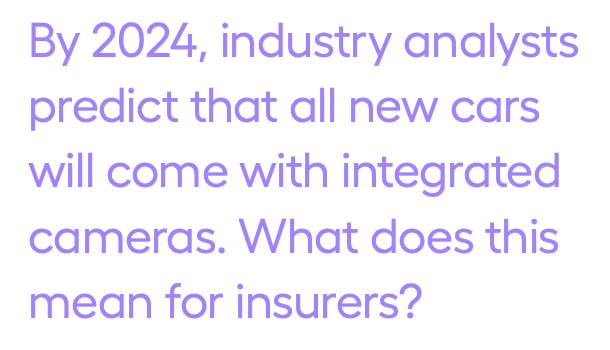
Vision is the ultimate sensor. It adds significant information on top of existing GPS and sensor data (e.g. accelerometers, telematics in general) and contains a lot of context about the road and any incidents in it. This means the creation of new forms of rich visual information about driving and all actors on the road, applicable to insurance and many other applications.
Who will own this data? Will it affect the relationship between drivers
and insurers? Will it change the claims process, policy underwriting
or both? How will the relationship between first and third parties to a collision change? Will UBI change too? Will the balance of power between insurers and automotive giants change? How about the relationship with technology giants whose operating systems will be embedded in these cars of the future?
To make better sense of this future, let’s examine several angles and understand the new technology landscape that is forming:
The Power of Vision Data
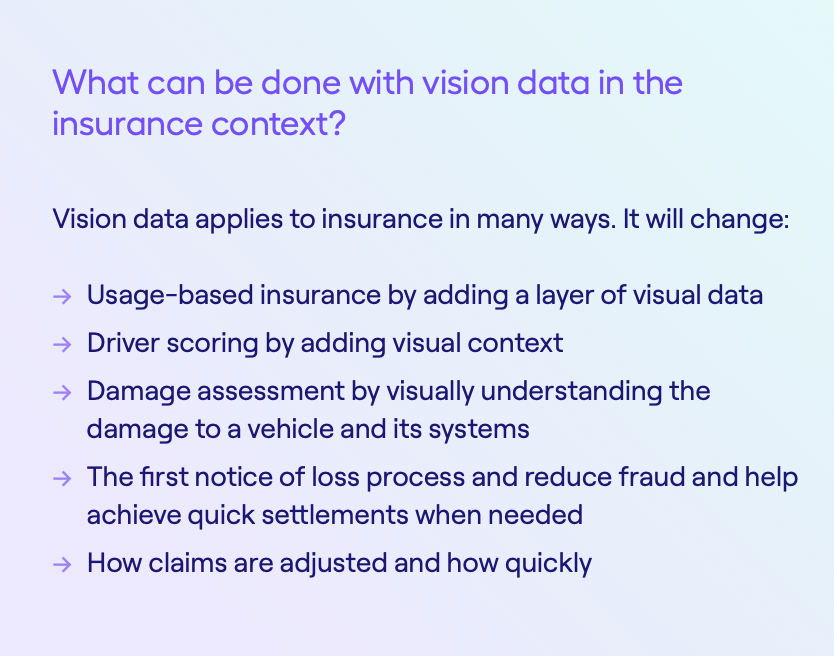
As stated above, vision data is always more powerful than other forms of data from the road. It’s the old adage about one picture being worth more than a thousand words (or a thousand hours of collision investigations). Vision data can be augmented with additional data, such as location or accelerometer data, for a deeper understanding of an event.
Vision data contains everything: the exact location of all agents on the road, from debris to potholes, pedestrians, bicycles and more. It shows driver behavior, from switching lanes to tailgating, and how all agents on the road interact with each other.
Sensor data is more limited. For instance, think about a stop sign or a pothole. You can “sense” that the car made a hard brake in front of a stop sign or pothole, but you can’t “see” the actual occurrence, only infer its existence, thus remaining uncertain whether this is a pothole or a stop sign. Similarly, a hard brake can indicate a car was tailgating another vehicle, but without the visual evidence, there is no way to positively know that this is indeed the case. In this respect, vision data, even today, is better and richer than sensor data, and can augment Usage Based Insurance as well as Driver Scoring (was the driver braking because they are careless
or because a child bolted into the road?). Vision gives us the ability to understand driver data in context, and in most cases, context is all that matters. All this applies to claims resolution and liability determination too, since video evidence “sees” all there is to see in a collision.
The Role Of Artificial Intelligence
Sometimes video evidence, raw and plain, with no AI, is all that’s needed for a good insurance outcome. We’re seeing this with small fleet insurers, where a simple video of an incident can quickly help determine whether a large payout is at risk, which might cause the insurer to settle as soon as possible. In this case, getting the small fleet (or trucker or rideshare driver) to drive with a dash cam is all that’s needed.
Yet sometimes combing through thousands of videos isn’t (humanly) possible. This is where vision-based Artificial Intelligence has a role to play. Simply put, we can use computers to see what humans cannot see.
AI can be used to understand how well drivers drive (not to be confused with driver assist, which would alert drivers but not necessarily score them, which would require another level of context), and whether they drive under certain risky conditions that can be changed.
Similarly, AI can scan hard brake videos to determine which one contains a real collision, thus creating an automated video FNOL. It can automatically reconstruct a collision, to understand what happened in it and speed up the claims adjustment process, as we’ll discuss below.
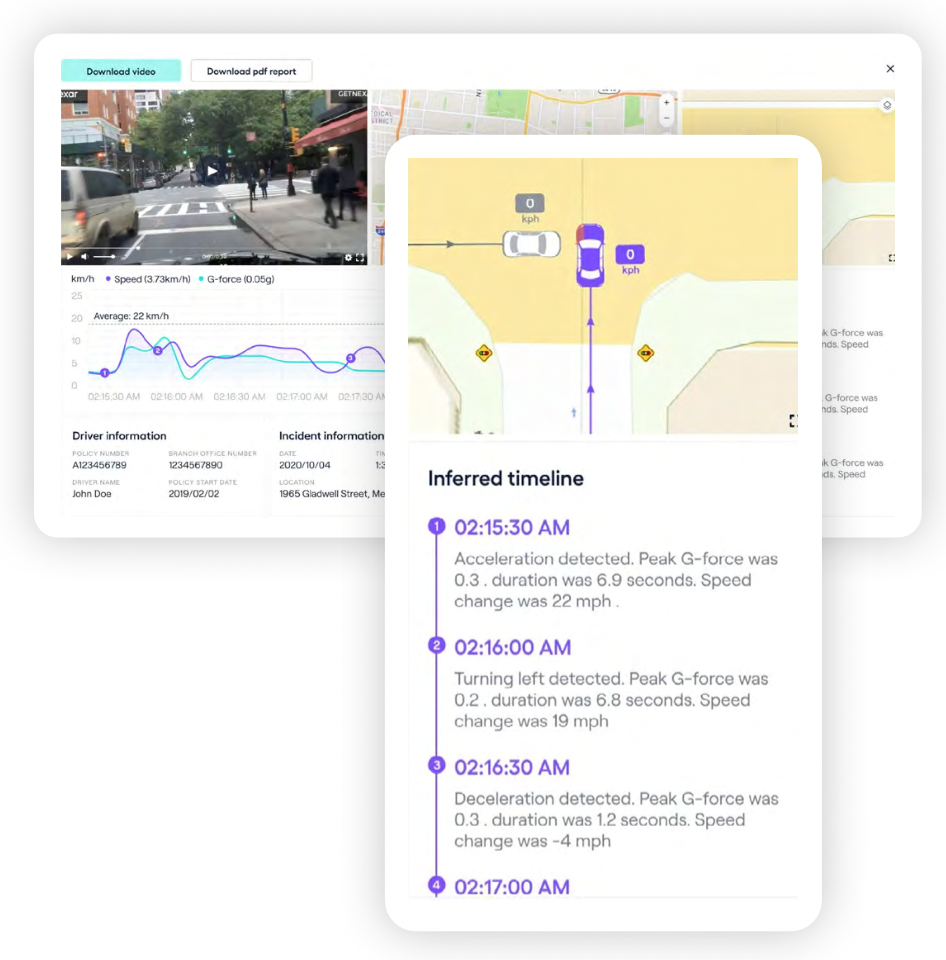
Video FNOL and Collision Reconstruction - how it works
Nexar has a deep learning pipeline that collects rare events like collisions and near-collisions from dash cams. After detecting such events, they are uploaded to the cloud and processed using multiple deep AI networks as well as a human-in-the-loop feedback for verification. These rare driving events are called “corner cases” and are used to train our AI models, so we can reconstruct collisions using AI.
As detection of corner cases can be very tricky, we developed a set of triggers that assist us in choosing the relevant scenarios for addition into our database. These triggers include:
- Vision-only triggers that detect pedestrians on the road (jaywalkers), vehicle cut-offs, cyclists that are on a drivers lane, or snow on the road.
- Vision and Inertial Motion Unit (IMU) sensor data - Detecting collisions, near-collisions and hard breaks. This is extremely important as IMU data is not enough for collision detection. By augmenting vision data with IMU data we can understand what is an actual collision vs. “just” a hit in the dash cam.
- Vision, IMU and mapping data - allows us to upload videos from areas we consider as challenging environments, such as construction zones, left turns, or road blockages. Such areas have a high probability for hard brakes or near collisions.
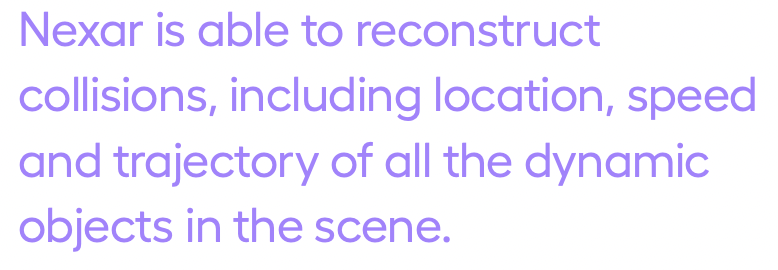
Nexar’s scenario reconstruction engine (used for insurance video FNOL and collision reconstruction) is trained against our corner cases dataset. These corner cases provide two main outputs: a bird’s-eye view of what happened including a trajectory of the collision, and a timeline of all the events in the video including vehicle speed, maneuvers, and the collision dynamics. Augmenting vision as part of these corner cases improves our understanding of the collision as well as the reconstruction of what happened.
As a result, Nexar is able to reconstruct collisions, including location, speed and trajectory of all the dynamic objects in the scene. In real time, this provides an FNOL function, and when claims are submitted, this speeds up the claims triage and adjustment process and unifies it. In the future an additional layer can be added, with information about the relative liability of the actors, based on traffic signs, lights, lanes, and a rule engine.
How Nexar’s Collision Reconstruction Works
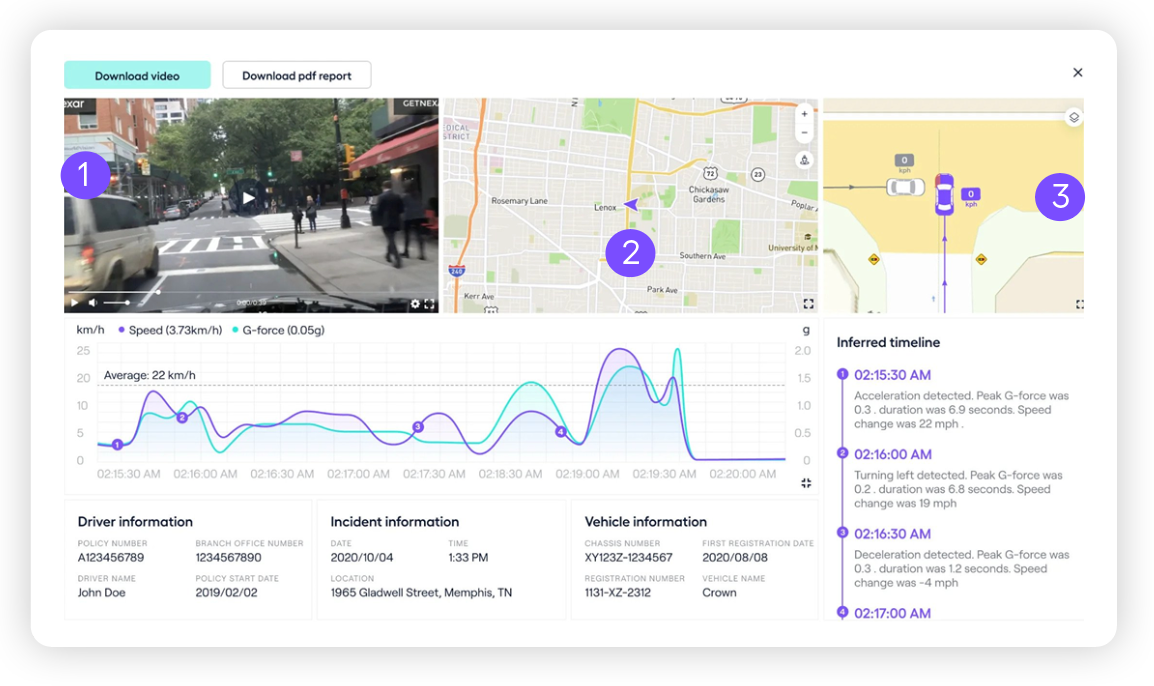
1. Detection - Supporting one-click reports, a video FNOL, with all information localized and in-context.
2. Collision Reconstruction (1st party) - A second-by-second timeline reconstruction with driver behavior (e.g. speed, distance and braking data) and a detailed status of signs and traffic lights
3. Scene Reconstruction (3rd party) - A detailed collision timeline that includes 3rd party behaviour, (e.g. speed and location), automatically providing full sensor and visual verification of what actually happened between colliding vehicles.
Conclusion
Nexar’s collision reconstruction is deployed in Japan with Mitsui Sumitomo Insurance (MSI) in over 250,000 connected dash cams. In New York, ride share drivers use Nexar dash cams and the video is used for evidence
in case of collisions. In Texas, our technology is used for video FNOL to quickly settle what can become “nuclear” loss verdicts.
Vision data from cars is on the verge of massive availability. Insurers need to take advantage of the immense potential inherent in the data to provide better customer service through quick claims resolution and better management of loss events, yet the potential of data from cars will be much bigger, giving better insight to make optimal underwriting decisions.