To make autonomous vehicles a reality, a change of course is needed
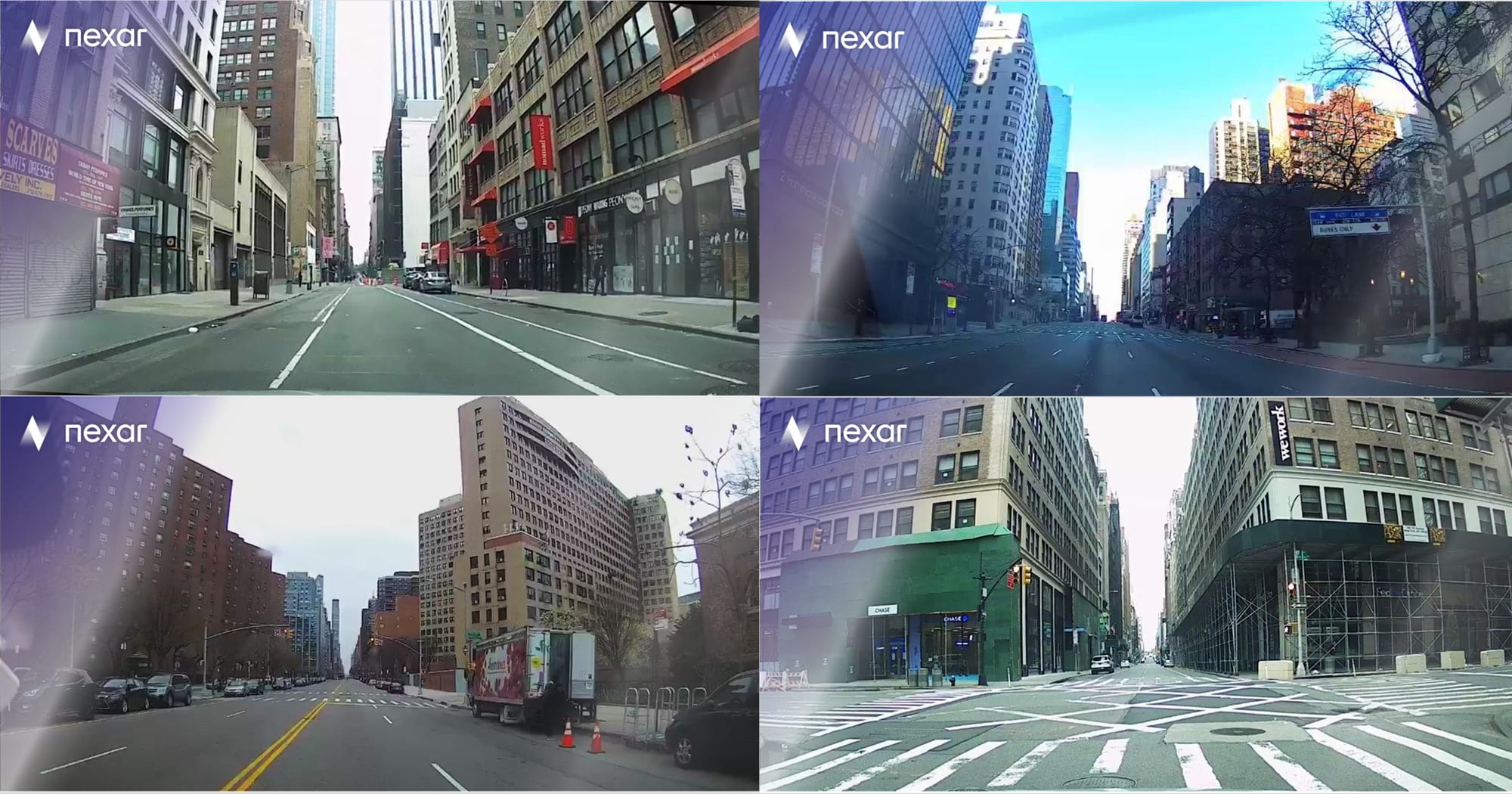
In 2015 the Guardian predicted that “In 2020, you’ll be a permanent backseat driver”. It is now 2021, and current predictions forecast many more years before we see this level of autonomous driving. Another thing is becoming evident, too: people expect AVs to drive better than humans, with no collisions. For all this to happen, the AV industry needs vision data for training. Lots of it.
Until the past year, conventional wisdom has it that the future of mobility depended on very expensive and accurate sensors, LiDARs, camera arrays and more. This resulted in small scale HD maps and not enough data to train AVs. Today, it seems that AV companies are inevitably running into issues with gathering enough data to replicate the totality of the human driving experience.
So what is missing on the data front for AV training?
Corner cases are what matters
One of the issues associated with deep learning for AVs is the problem of data scarcity. There are many cases of “straight forward” driving but, in fact, what AVs need are the near miss and collision incidents that unfortunately all of us have experienced. Be that a pedestrian crossing unexpectedly, a collision ahead of you on the highway, or any near-collision, AVs need the data about how that problem was overcome by a human driver.
Data scarcity means, in this context, that the more extreme cases aren’t available for training. The term for these occurrences is “corner” or “edge” cases - when some variables are completely outside what’s considered “normal”. Thing is, for safe AVs, AVs need to know what to do with these corner cases and those aren’t available.
The reason behind this lack of data is that AVs are expensive vehicles, and don’t drive enough miles to create all those corner cases. They are also driven in lower risk environments: in states with few weather issues, and away from road zones and road closures, which are very confusing to an AV.
Issues with the availability of collision information made this problem worse. It is almost impossible to know how many collisions happen in a given metro area, especially with regards to those collisions that have a huge impact on lives and safety, but aren’t tracked by the police or insurance companies. These are near collisions or low g-force ones. In order to train well, AV training needs to see them all. Instead, AV companies were using police reports to reconstruct collisions. This lack of data also impacts the safety argument, since you can’t compare the safety of AV driving to the safety of human driving, if you’re not sure what is the safety in terms of collisions and near collisions per miles driven for a human driver.
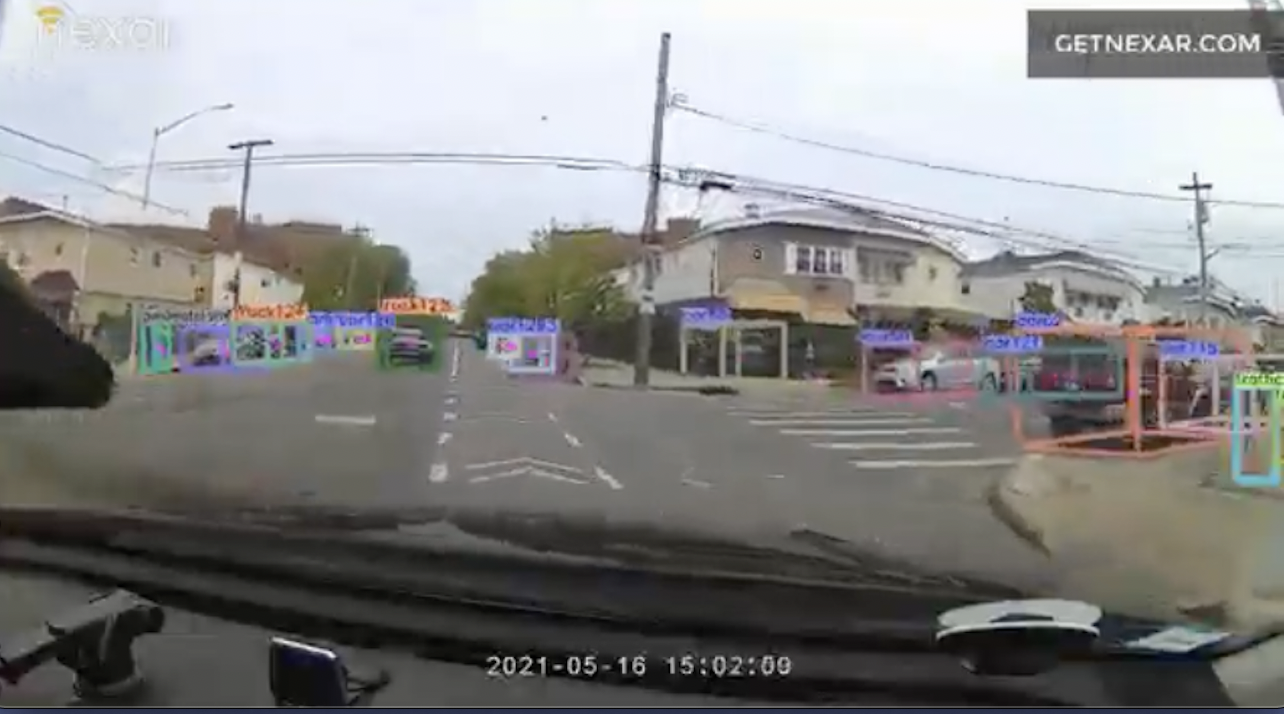
Cheap and at scale vs expensive
This is where the bottoms-up, crowd-sourced, cheaper approach comes into play. Instead of collecting data from dedicated vehicles with expensive technology, this approach takes the opposite route. It uses human drivers that already need a vision device in their car (this can be ADAS, dash cams or even driver monitoring systems) to collect cheaper data with low cost consumer devices. This approach isn’t better because it is cheaper. It’s better because it collects corner cases at scale, sees all near collisions (and what driver responses are) and is able to accurately answer the question of how many collisions and near collisions occur per driver mile. It is also the only way to begin to assess and train for the long tail diversity of edge cases. AV companies are expected to train without causing human casualties, and as a result don’t see these events in dangerous places, uncertain routes (impacted by work zones, for instance) and driving that is affected by inclement weather conditions.
Mapping needs crowdsourcing too
Mapping for AVs is undergoing a similar change.
HD Maps need to be kept up-to-date, and this can’t happen at a scale that ensures coverage and freshness for those maps, due to the high cost of keeping these maps updated with transient data. Transient data cannot be collected and analysed at scale with the unit economics of LIDAR or pure video. We need crowd-produced and edge-reduced data.
We believe the correct approach is distributed AI and network optimization that selects the right video and images seen by the network of cameras while keeping the connectivity budget down. This requires vehicle positioning, object detection and localization to be able to create a layer of transient map data using commodity cameras.
This approach creates a new world, dominated by cameras that are already deployed with consumers, which can create the AV training information needed and provide the roadmap to the right freshness of maps that will deliver on better mobility and a collision free world.