AI is Slashing the Cost of Making Maps - Here's Why
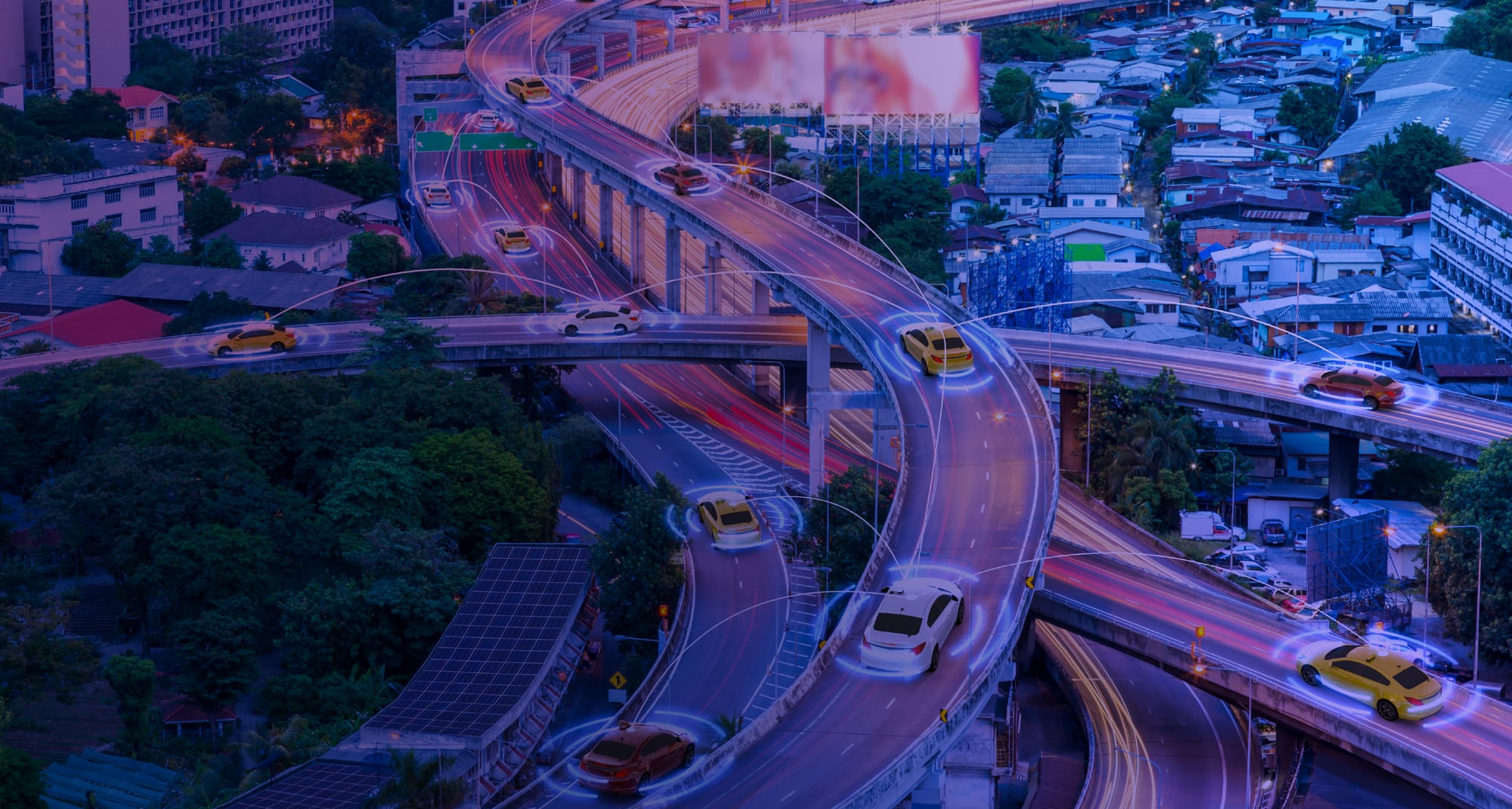
The cost of making maps in real time is dropping rapidly, thanks to a new generation of crowdsourcing and edge AI technologies. These technologies enable connected vehicles and dash cams to create fresh data-rich maps automatically from the road without human intervention. In this essay, we'll take a look at how edge AI and crowdsourcing are revolutionizing the mapmaking industry, and why now is the time for people, organizations and businesses to leverage this transformative technology.
1. Traditional map making is slow and expensive
Although maps have quickly become digital, map making itself is still largely dependent on manual processes from the past. This includes using a dedicated mapping fleet to survey the roads at high cost (and therefore infrequently), buying and merging data from different public and private sources, and paying for thousands of human workers to verify their data sets. Even in our fast paced world, manual annotation of maps can take many months and be prohibitively expensive - not to mention highly outdated by the time the work is actually finished. Recent advancements in aerial imagery notwithstanding, the manual processes used to update and maintain geospatial data leave traditional map making far behind the technological advancements of searching the World Wide Web or generating human-like text with ChatGPT.
To understand why traditional map mapping is so expensive, consider the following example. To reach 100 visits per mile in a month, a city like Phoenix, Arizona with 5000 miles of roads would need to employ a dedicated mapping fleet of 360 vehicles. For the entire United States, the required fleet size would be 180,000 vehicles. With a Lidar equipped car costing around $1.5M each and mapping costs reaching $30 per mile per car, it is no wonder that autonomous vehicle companies are paying tens of millions of dollars to cover a fraction of the US territory.
Maps are often wrong and outdated, because they are built more like inventories, structured lists of things that are pinned to the real-world via longitude and latitude with a semantic layer of connectivity. With fragmented data, scattered over different silos from city halls to highway contractors and mapping service providers, it becomes difficult if not impossible to obtain a complete data set depicting the ground truth that people, vehicles and fleets actually need.
The extraordinarily high costs of traditional mapping have caused the market dynamics to be such that only a handful of companies actually collect and process mapping information. This includes companies like Here and Tomtom who must toil to stay profitable, but also some of the largest companies in the world like Apple and Google. As we will show next, the new mapping regime will cause the cost of mapping to plummet and likely create a Cambrian explosion of companies, services and use cases. These will all of a sudden become financially viable, thereby creating a new market for real-time location-based services.
Whereas the human-centric maps of incumbent map providers will likely still serve us for a number of years, the software-centric maps that will save lives, support seamless mobility and delight customers can only come from this radically new method to collect, process and distribute road information in a fast and scalable way. This revolutionary step is real-time mapping powered by edge AI and crowdsourcing.
2. The solution is Real-Time Mapping with Crowdsourcing AI
The way to leverage AI to its full potential and build a cost-effective real-time mapping platform is to spin the flywheel in Figure 1 as follows:
- access the images and videos from millions of vehicle cameras (“more eyes on the road” and “more coverage”),
- use AI at the edge to detect and aggregate all relevant road objects, features and changes, at a fraction of the cost of a cloud-only solution (“better data”),
- build AI driven 3D reconstructions that eliminate the need for annotation and locate all changes with an accuracy of tens of centimeters,
- deliver powerful, easy-to-use APIs to deliver the real-time mapping data that other companies and software developers need in order to build the next generation of mobility products and services (“better apps & services” and “more demand”).
Figure 1 The flywheel for real-time mapping.
By leveraging the power of crowdsourcing and utilizing edge AI technology, real-time mapping is generated automatically from billions of images that are captured and processed locally by moving vehicles and dash cams. Vehicle cameras are ideal because the data source can be verified and trusted, plus no other sensor gives as much information gain at such low cost. By turning every vehicle effectively into a mapping vehicle, this massive network dramatically reduces the need for costly data gathering, complex hardware and manual annotation, while finally delivering the high levels of coverage, freshness and precision required by modern mobility services and applications.
3. Reducing the unit cost of real-time mapping by 40x
In order to retrieve mapping information we must first need to collect it at an affordable unit cost. As shown in Figure 2, Nexar's CityStreamTM platform achieves this goal through the combination of five main innovations:
- Crowdsourcing road data: CityStreamTM leverages consumer dash-cams, commercial fleets, mobility as a service (MaaS) providers, auto OEMs and autonomous vehicles as “eyes on the road” allowing for unprecedented density and coverage — at a fraction of the cost of dedicated mapping fleets. This network of “road crawlers” not only enhances precision by enabling multiple witnesses to validate the acquired road data in real time, but also caps the cost dramatically by transferring to the cloud only the delta difference between visits. An additional CityStreamTM feature allows users to tune the detector flexibly to the required level of precision and computation.
- Edge AI: This includes both the consumer edge (vehicle cameras) and the provider edge (local infrastructure). The Nexar SDK, which is installed in dashcams (and companion smartphone apps), vehicle cameras and multi-access edge computing (MEC) devices, enables CityStreamTM to run AI models, detections and aggregation algorithms at the edge of the network. This form of distributed AI reduces the cost of transferring unnecessary images to the cloud, while increasing the trust that comes from making local decisions on what each camera is actually seeing. From a computational perspective, CityStreamTM is also crowdsourcing the distributed storage and processing hardware that is required to realize the promise of real-time mapping.
- Change detection: Instead of sending all detections to the Cloud at a very high cost, Nexar’s SDK is also able to make local decisions on what is different and what remains the same in every road. By concentrating on the much smaller set of differences (from what was mapped), change detection reduces even more dramatically the amount of data to be transferred, stored and processed in the Cloud.
- Frame on demand (FoD): Applications and services are given the option to fetch image frames just in time, based on the timestamps and georeferenced locations. This feature, which can be used for evidence-on-demand in the form of single images to short videos, is best achieved in a self-regulating dynamic manner, so as to further control the unit costs of real-time mapping.
- 3D HD map reconstruction: A new generation of automated HD maps leverages 3D reconstruction from massive data sets of road images and achieves an accuracy of tens of centimeters without human annotation.
Figure 2 The CityStreamTM platform implements all necessary functions and key APIs for real-time mapping.
This combination of automated crowdsourcing with real imagery captured intelligently at the edge and delivered as value-added data products via cloud APIs enables Nexar to play a singular role in dramatically reducing the cost of mapping each mile and bringing real-time mapping to reality. Case in point, in Phoenix Arizona 54% of the road information was incorrect or not even available in the Open Street Map (OSM) when compared against what the Nexar network was able to detect automatically.
4. What real-time mapping means for people, vehicles and businesses
When you take a deeper look at the practical use cases of AI crowdsourced road data, it’s actually way more than just maps. Road signs that change from one day to another, work zones that block traffic for a few hours, parking spaces that become free this minute or collisions that happen in a second are just a few examples of life happening in real time. And the network sees it all. Whereas humans are able to handle incomplete data and project from a two-dimensional representation to the actual real world, today's software-defined vehicles need fresh, precise mapping information and way more contextual data. Without it, they are unable to achieve the situational awareness that is necessary to assist the driver reliably let alone take over the steering wheel.
The key to unlock the benefits of real-time mapping is to identify what data you will use for your purpose and determine the value this brings to your business. A typical analysis includes:
- Freshness: How often you need to get each type of data, i.e. monthly, weekly, daily, hourly, minute-by-minute.
- Geography: What coverage you need to have, i.e. district, highway, city.
- Data format: Which types of data, such as locations, images, videos, etc. and in what format.
- Object type: Work zones, road signs, potholes, intersections, etc.
- Curation: How curated the data must be, e.g. Raw, 10% mis-detections, human-verified.
- Interface: How you wish to consume the data, eg. via APIs, online map, dashboard, visualizer, etc.
5. Final Thoughts
By building a massive network of “eyes on the road” and bringing AI to the network’s edge, Nexar has built a large-scale high-performance platform that crowdsources more than 20 Million detections per day, builds new real-time maps and delivers petabytes of visual data from hundreds of thousands of vehicle cameras — all at a cost that the industry can actually afford. Road signs, variable speed limits, collisions, cars stopped on the shoulder, parking, weather phenomena, lane markings and bike lanes are only a few of the use cases that make a difference when moving people and goods. By using Nexar CityStream APIs and/or SDK, all industry players can finally leverage edge AI and the power of real-time mapping to improve comfort, increase safety and build the next generation of mobility solutions.
If you are interested in discovering more about real-time mapping with CityStream Live, download this whitepaper or try for yourself here.