How car camera applications can help us understand the cost differences for endpoint, edge or cloud AI/compute
In the next 3–5 years, all new cars will contain cameras. Several cameras per car, actually. Will car cameras remain confined to driving assist functions, or will they evolve into a new way of seeing and sensing the roads and the world around them?
The question of what can be done with car camera output is crucial. Vision data can offer a strategic and competitive advantage, from enabling novel (and revenue generating) driver apps to forming a strategic vision data asset that can transform cities, mapping, delivery, public transit and more.
Vision is different
Today, virtually every sensor and component in the car is “connected”, meaning sent outside the car. An awful lot of telemetry data is becoming available, from car data attributes that specify location, engine status, speed, maintenance, and even if a door was locked or the car refueled. This data comes from the vehicle’s electronic control units (ECUs), Controller Access Networks (CANs), and even infotainment systems. Vision data, from the various cameras in the car can provide far better “sensing”, creating the eyes on the road that drivers need for better driving, safety and security.
For instance, think of an application that uses crowd-sourced vision (vision coming from a “shared memory” of the cars surrounding you) to indicate a free-parking spot that you can see on a map and get driving directions to, including telling you when that free parking spot was just seen. Using telemetry data, you could probably detect 3–4 parking spots in a day, and it would probably be difficult to infer whether those parking spaces are indeed legitimate (since some vision data, such as parking signs, road markings etc aren’t available). With vision, the uber sensor, you can detect about 100 free parking spots in one hour and use AI and vision to ensure that the spots detected are legitimate.
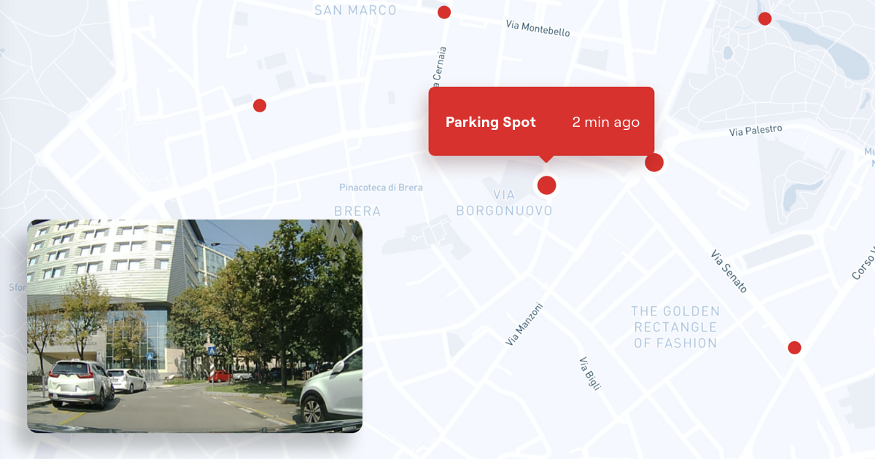
Other examples of ultra efficient AI models that work today are a construction zone detector, that sees whether there are road encroachments as a result of building or road work activity and provides that data to drivers and cities.
Yet today car camera can’t be connected as simply as telemetry data. Video is heavy, and requires both compute and bandwidth, both of which can come with a considerable price tag. As a result, in many cases car camera information remains on an SD card in the car or is just thrown to the wind.
But if we solve these issues, a whole new world of capabilities gets unlocked. We could bring to market new driver centric services, powered by an online network of all-seeing eyes, and turn the output of cameras into a massive asset for automotive OEMs and the drivers that they serve.
Edge AI can and will enable vision
So, if transporting car camera vision data and running AI on it are essential, how can this cost barrier be overcome? The answer lies in edge AI — the ability to run efficient AI models at the network edge, such as 5G networks, or on the device/vehicle endpoint itself.
Before we explain how this is done, it’s important to first understand three elements to consider with regards to where compute can and should happen:
- Model complexity: is the model simpler (such as object detection) or is it more complex, requiring a situational understanding or multi sensor aggregation? The more complex the model, the less likely it is to run on the endpoint.
- The connectivity budget: is it restricted (less than 0.5 Gbs per month), unlimited or somewhere in between? This impacts the selectivity of data transfer — what type of data cherry picking occurs from the device to the cloud.
- The latency tolerance of the specific application: is it mission critical (such as a safety alert based on crowd-sourced vision) or not (for instance an image of the street for mapping purposes), or something in between such as a free parking spot.
This means that the right answer for edge AI isn’t “how can you do it on the edge” but rather that an underlying system is required: a way to optimize your compute and connectivity “budgets” with the specific application in mind. First, by making the right decisions as to what data to upload, cherry picking the right information to send (and providing privacy and anonymization too), and second, by making decisions as to where to run compute, based on the value, function and timeliness of the detections.
Putting a $$ value to the different compute options
Can you really put a dollar value to the different options: edge, endpoint and cloud compute?
You can.
We ran a hypothetical model of crowd sourced vision for one million vehicles, based on both capex and opex. As you can see, running a vision use case in the cloud would cost 42 X times the cost of implementing the same use case at the edge, taking into account connectivity and compute costs. Edge compute is 29 X the cost of device inference. At the same time, it’s important to point out the tradeoff of capabilities, since not all AI can run in each of the options. That is why the optimal platform should be holistic and adaptive, allowing use cases to leverage edge AI, but also to allow for aggregation and other functions to run in the cloud, where a fuller perspective is achievable.
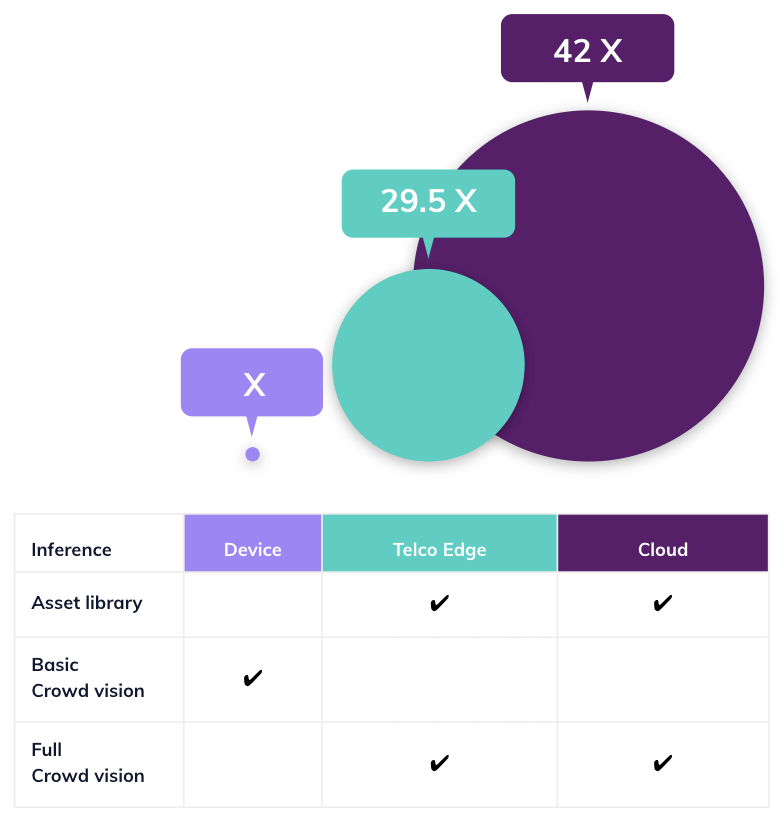
And perhaps the main issue isn’t edge vs cloud compute, but rather that the scale-proof and future-proof architecture for building a massive data data asset is to turn the vehicles themselves into compute nodes, and to use them as repositories, allowing for decentralized indexing and access, allowing for cherry picking, dynamic AI models implementation, and more.