Do Cities Need Fresh Images For Better City/Road Management?
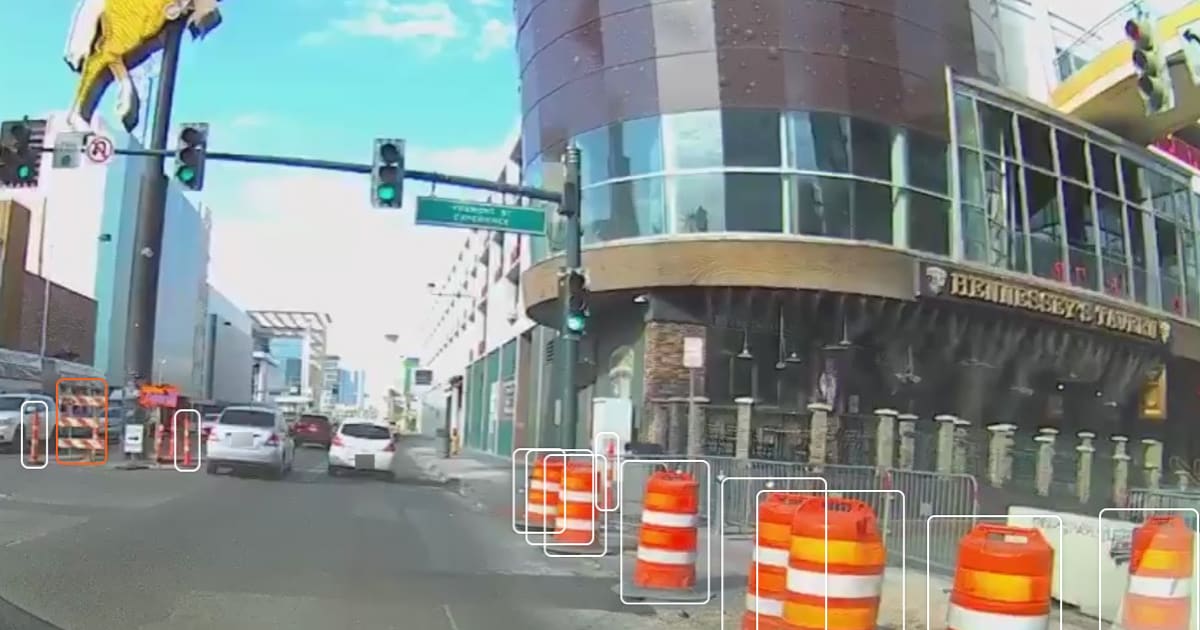
Can artificial intelligence do the job?
When cities need to make decisions about managing assets, streets, permits, they need to be able to see what is actually going on. Some of this depends on actual human sight, such as looking at a road, assessing damage, or checking whether a work zone actually complies with permit requirements. However, deploying humans or physical cameras to see what’s going on doesn’t always make a lot of sense from an efficiency perspective.
Nexar’s dash cam network can be used to pull imagery of the streets and assets in the public space and help cities and road and transportation officials do a better job by unleashing vision data that they need. Using this data, cities can use a recent image stream of roads, road inventory and streets, better manage road inventory and roads while using intelligent infrastructure to see and monitor lane blockages and road work zones.
What do cities do today when they need to examine something?
We actually asked just that of city and transportation officials during one of our webinars. Here are their responses:
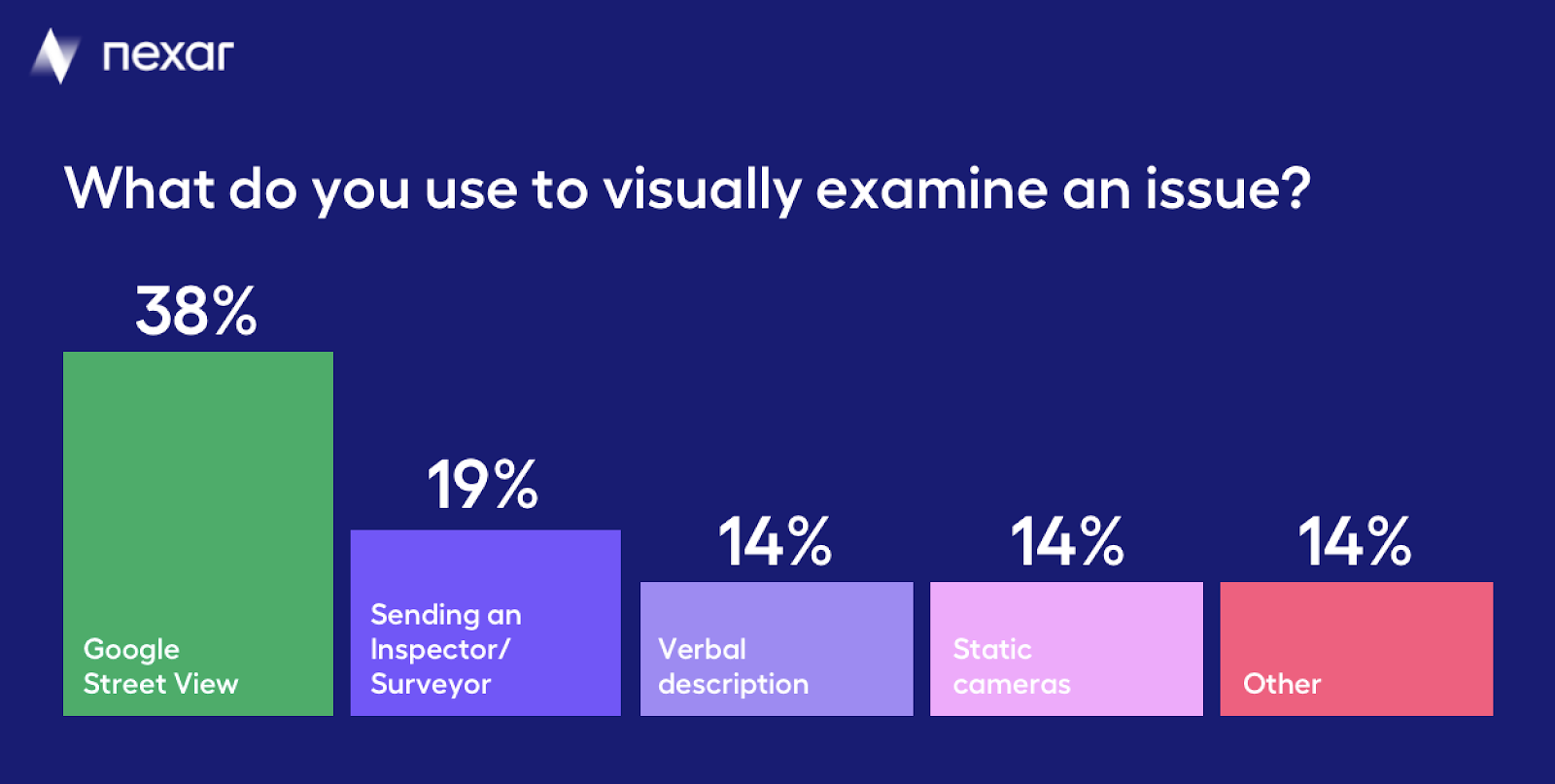
As you can see, only 14% use a fresh source of imagery (static cameras), and the rest rely on other sources, from somewhat stale Google StreetView imagery to verbal or manual inspection.
Don’t we have enough cameras on the streets?
Cities have been investing in cameras in many public spaces, mostly as part of safety initiatives. These video feeds are used to look for missing persons, criminal activity and to understand the state of the city. Typically, no artificial intelligence is deployed on top of these vision streams, meaning that people actually fast-forward or rewind to find what they are looking for. In most cases, the installation of the cameras is reserved for high traffic areas, and they do not provide broad coverage (we’ll refer to the privacy questions this practice raises below).
Cities also “see” the roads using other methods, such as through the use of commercially available datasets (mobility, telemetry and even surveys). Obviously, physical cameras on poles and traffic lights and data sets are useful. However, they aren’t transformational, in that the entire geography of a city or road isn’t covered, and, the costs involved with such methods can be substantial, making blanket coverage impossible.
Dash cams can actually provide blanket coverage and typically, since the images come from drivers using them as they drive (for insurance purposes), there is no effort required to stand up a network.
Images, detections or changes? What is Artificial Intelligence for?
Artificial intelligence is quite useful when one looks to make use of road imagery. It is used to accurately locate images, even in dense urban canyons where GPS information loses its accuracy (you can read about this here). It is also used to select which images are most important. But, in a general sense, one can think of the options coming from a vision data product, as the following, all dependent on the degree of the use of AI:
- Imagery: in this case, the vision data is images — of a building, a street, pedestrians. Images can be quite useful, since they help “seeing” what citizens see and understand the state assets are in. We’ve seen cities use imagery to understand roadway topography, turn regulations, and buildings. Where images aren’t useful is when you need to see something specific and you don’t want to scroll through endless images- such as all stop signs in a city. This is where detections come into play.
- Specific detections: detections can be all road signs, all traffic lights, or more complex detections such as a work zone detection (consisting of barrels, diamond signs etc). Detections are quite effective to understand substantial blockages (and whether they are in compliance with a permit) since they save the labor of actively looking for a blockage but rather immediately point them out. Other detections, based on a combination of mobility and sensor data as well as vision data are hotspots and places where people speed or slow down, such as potholes.
- Change detection: In this case, AI is used to not look for a specific element (such as a work zone) but rather changes, such as store sign changes, differences in tree canopies etc.
All in all, the use of AI depends on the specific application required.
What exactly would you like to see? Other considerations
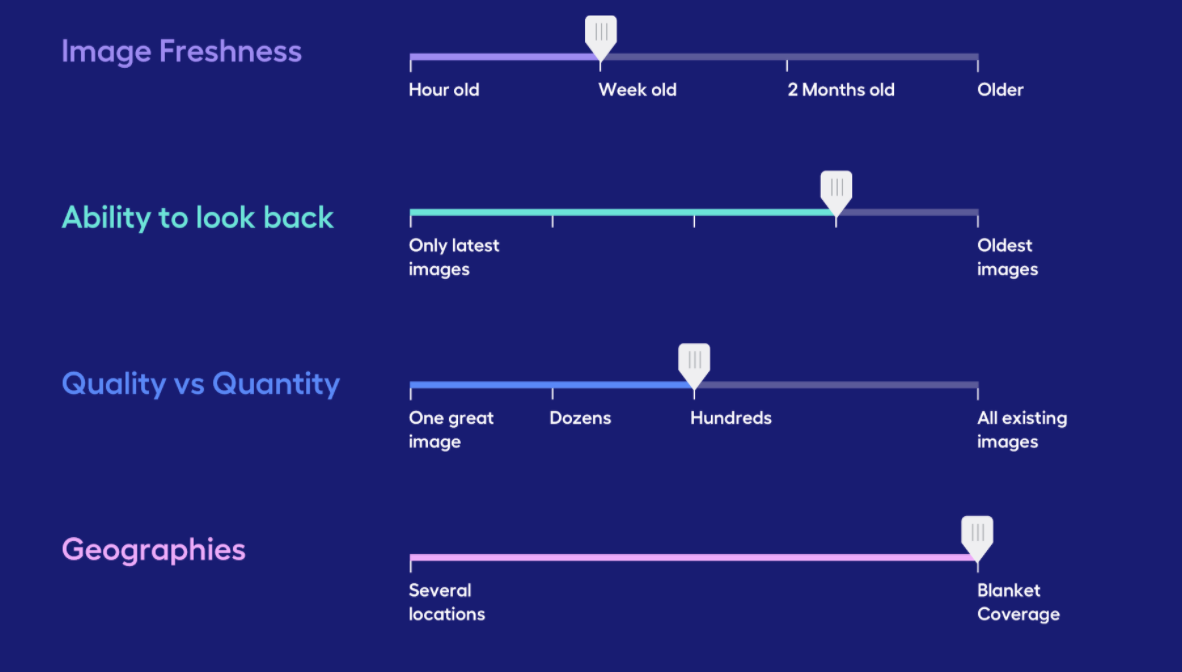
Saying that cities need to “see” isn’t enough — you need to also define what exactly needs to be seen, even after you’ve made the choice of image/detection/change detection. Here are some additional considerations:
- Image Freshness: what is the age of the image that is required? For road inventory detections, images that are a month old should be enough. Yet, for work zone detections, daily image freshness is probably better. In other cases, images that are a few hours old may be required, perhaps to monitor a developing situation in a busy area.
- Ability to look back in time: sometimes all that is needed is a snapshot in time, other times the need is to understand the same location across many points in time. An example can be a city street — looking at pedestrian activity over a period of time and in many different times of day.
- How many images are required (quality vs quantity): Another question is whether one “perfect” image is required — or whether many images need to be reviewed, saving the cost of using AI for the perfect image and perhaps creating more insight for the reviewer. This of course depends on the use case.
- Coverage: last but not least, there is a question of coverage — how many images are required and in which areas of the city — main arteries vs residential areas etc.
When we surveyed city officials — here are their responses with regards to image freshness:
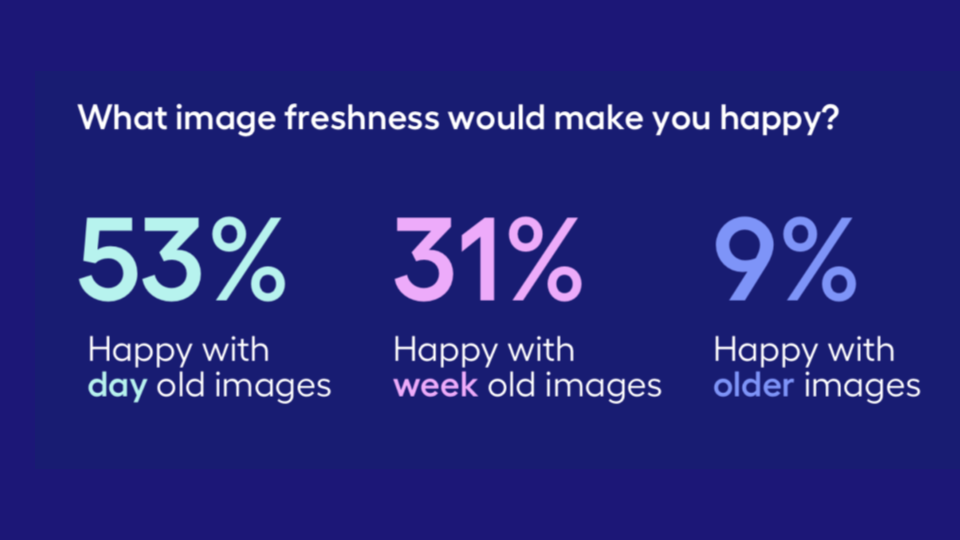
Privacy — an important note
One of the differences between fixed cameras and the use of dash cam imagery is privacy. In many cases, dash cams on public vehicles (buses, garbage collection) are used for enforcement (e.g. fining cars that use dedicated bus lanes which isn’t allowed by local regulations). The same applies for cameras on traffic lights. Dash cam imagery, though, is entirely anonymized and contains no PII (Personally Identifiable Information), so that uses are always around understanding and managing the physical world (except in cases where work zone permit compliance is checked, but then it’s about the work zone permit holder). In short, dash cam imagery, or car-sourced imagery is about places and not people.
So, what can you do today with imagery of your city?
You can begin with a simple view of the dash cam imagery that already exists in your city, and see which use cases it fits. Accessing Imagery is as easy as using Google StreetView. Initially, you will probably focus on a limited geographical area. As the use cases and locations where imagery data become evident, you can focus on increasing coverage and using detections — road inventory and work zones, perhaps. You could also integrate the inventory into a visual verification of complaints coming into 311. You can see what’s going on in your city, remotely, and the way to do so is to unleash the data that car cameras collect.